Author: Jason Brownlee
Training a neural network with a small dataset can cause the network to memorize all training examples, in turn leading to poor performance on a holdout dataset.
Small datasets may also represent a harder mapping problem for neural networks to learn, given the patchy or sparse sampling of points in the high-dimensional input space.
One approach to making the input space smoother and easier to learn is to add noise to inputs during training.
In this post, you will discover that adding noise to a neural network during training can improve the robustness of the network, resulting in better generalization and faster learning.
After reading this post, you will know:
- Small datasets can make learning challenging for neural nets and the examples can be memorized.
- Adding noise during training can make the training process more robust and reduce generalization error.
- Noise is traditionally added to the inputs, but can also be added to weights, gradients, and even activation functions.
Let’s get started.
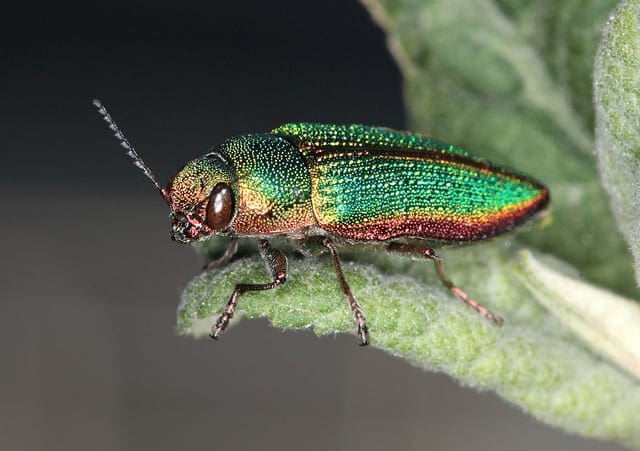
Train Neural Networks With Noise to Reduce Overfitting
Photo by John Flannery, some rights reserved.
Overview
This tutorial is divided into five parts; they are:
- Challenge of Small Training Datasets
- Add Random Noise During Training
- How and Where to Add Noise
- Examples of Adding Noise During Training
- Tips for Adding Noise During Training
Challenge of Small Training Datasets
Small datasets can introduce problems when training large neural networks.
The first problem is that the network may effectively memorize the training dataset. Instead of learning a general mapping from inputs to outputs, the model may learn the specific input examples and their associated outputs. This will result in a model that performs well on the training dataset, and poor on new data, such as a holdout dataset.
The second problem is that a small dataset provides less opportunity to describe the structure of the input space and its relationship to the output. More training data provides a richer description of the problem from which the model may learn. Fewer data points means that rather than a smooth input space, the points may represent a jarring and disjointed structure that may result in a difficult, if not unlearnable, mapping function.
It is not always possible to acquire more data. Further, getting a hold of more data may not address these problems.
Add Random Noise During Training
One approach to improving generalization error and to improving the structure of the mapping problem is to add random noise.
Many studies […] have noted that adding small amounts of input noise (jitter) to the training data often aids generalization and fault tolerance.
— Page 273, Neural Smithing: Supervised Learning in Feedforward Artificial Neural Networks, 1999.
At first, this sounds like a recipe for making learning more challenging. It is a counter-intuitive suggestion to improving performance because one would expect noise to degrade performance of the model during training.
Heuristically, we might expect that the noise will ‘smear out’ each data point and make it difficult for the network to fit individual data points precisely, and hence will reduce over-fitting. In practice, it has been demonstrated that training with noise can indeed lead to improvements in network generalization.
— Page 347, Neural Networks for Pattern Recognition, 1995.
The addition of noise during the training of a neural network model has a regularization effect and, in turn, improves the robustness of the model. It has been shown to have a similar impact on the loss function as the addition of a penalty term, as in the case of weight regularization methods.
It is well known that the addition of noise to the input data of a neural network during training can, in some circumstances, lead to significant improvements in generalization performance. Previous work has shown that such training with noise is equivalent to a form of regularization in which an extra term is added to the error function.
— Training with Noise is Equivalent to Tikhonov Regularization, 2008.
In effect, adding noise expands the size of the training dataset. Each time a training sample is exposed to the model, random noise is added to the input variables making them different every time it is exposed to the model. In this way, adding noise to input samples is a simple form of data augmentation.
Injecting noise in the input to a neural network can also be seen as a form of data augmentation.
— Page 241, Deep Learning, 2016.
Adding noise means that the network is less able to memorize training samples because they are changing all of the time, resulting in smaller network weights and a more robust network that has lower generalization error.
The noise means that it is as though new samples are being drawn from the domain in the vicinity of known samples, smoothing the structure of the input space. This smoothing may mean that the mapping function is easier for the network to learn, resulting in better and faster learning.
… input noise and weight noise encourage the neural-network output to be a smooth function of the input or its weights, respectively.
— The Effects of Adding Noise During Backpropagation Training on a Generalization Performance, 1996.
How and Where to Add Noise
The most common type of noise used during training is the addition of Gaussian noise to input variables.
Gaussian noise, or white noise, has a mean of zero and a standard deviation of one and can be generated as needed using a pseudorandom number generator. The addition of Gaussian noise to the inputs to a neural network was traditionally referred to as “jitter” or “random jitter” after the use of the term in signal processing to refer to to the uncorrelated random noise in electrical circuits.
The amount of noise added (eg. the spread or standard deviation) is a configurable hyperparameter. Too little noise has no effect, whereas too much noise makes the mapping function too challenging to learn.
This is generally done by adding a random vector onto each input pattern before it is presented to the network, so that, if the patterns are being recycled, a different random vector is added each time.
— Training with Noise is Equivalent to Tikhonov Regularization, 2008.
The standard deviation of the random noise controls the amount of spread and can be adjusted based on the scale of each input variable. It can be easier to configure if the scale of the input variables has first been normalized.
Noise is only added during training. No noise is added during the evaluation of the model or when the model is used to make predictions on new data.
The addition of noise is also an important part of automatic feature learning, such as in the case of autoencoders, so-called denoising autoencoders that explicitly require models to learn robust features in the presence of noise added to inputs.
We have seen that the reconstruction criterion alone is unable to guarantee the extraction of useful features as it can lead to the obvious solution “simply copy the input” or similarly uninteresting ones that trivially maximizes mutual information. […] we change the reconstruction criterion for a both more challenging and more interesting objective: cleaning partially corrupted input, or in short denoising.
Although additional noise to the inputs is the most common and widely studied approach, random noise can be added to other parts of the network during training. Some examples include:
- Add noise to activations, i.e. the outputs of each layer.
- Add noise to weights, i.e. an alternative to the inputs.
- Add noise to the gradients, i.e. the direction to update weights.
- Add noise to the outputs, i.e. the labels or target variables.
The addition of noise to the layer activations allows noise to be used at any point in the network. This can be beneficial for very deep networks. Noise can be added to the layer outputs themselves, but this is more likely achieved via the use of a noisy activation function.
The addition of noise to weights allows the approach to be used throughout the network in a consistent way instead of adding noise to inputs and layer activations. This is particularly useful in recurrent neural networks.
Another way that noise has been used in the service of regularizing models is by adding it to the weights. This technique has been used primarily in the context of recurrent neural networks. […] Noise applied to the weights can also be interpreted as equivalent (under some assumptions) to a more traditional form of regularization, encouraging stability of the function to be learned.
— Page 242, Deep Learning, 2016.
The addition of noise to gradients focuses more on improving the robustness of the optimization process itself rather than the structure of the input domain. The amount of noise can start high at the beginning of training and decrease over time, much like a decaying learning rate. This approach has proven to be an effective method for very deep networks and for a variety of different network types.
We consistently see improvement from injected gradient noise when optimizing a wide variety of models, including very deep fully-connected networks, and special-purpose architectures for question answering and algorithm learning. […] Our experiments indicate that adding annealed Gaussian noise by decaying the variance works better than using fixed Gaussian noise
— Adding Gradient Noise Improves Learning for Very Deep Networks, 2015.
Adding noise to the activations, weights, or gradients all provide a more generic approach to adding noise that is invariant to the types of input variables provided to the model.
If the problem domain is believed or expected to have mislabeled examples, then the addition of noise to the class label can improve the model’s robustness to this type of error. Although, it can be easy to derail the learning process.
Adding noise to a continuous target variable in the case of regression or time series forecasting is much like the addition of noise to the input variables and may be a better use case.
Examples of Adding Noise During Training
This section summarizes some examples where the addition of noise during training has been used.
Lasse Holmstrom studied the addition of random noise both analytically and experimentally with MLPs in the 1992 paper titled “Using Additive Noise in Back-Propagation Training.” They recommend first standardizing input variables then using cross-validation to choose the amount of noise to use during training.
If a single general-purpose noise design method should be suggested, we would pick maximizing the cross-validated likelihood function. This method is easy to implement, is completely data-driven, and has a validity that is supported by theoretical consistency results
Klaus Gref, et al. in their 2016 paper titled “LSTM: A Search Space Odyssey” used a hyperparameter search for the standard deviation for Gaussian noise on the input variables for a suite of sequence prediction tasks and found that it almost universally resulted in worse performance.
Additive Gaussian noise on the inputs, a traditional regularizer for neural networks, has been used for LSTM as well. However, we find that not only does it almost always hurt performance, it also slightly increases training times.
Alex Graves, et al. in their groundbreaking 2013 paper titled “Speech recognition with deep recurrent neural networks” that achieved then state-of-the-art results for speech recognition added noise to the weights of LSTMs during training.
… weight noise [was used] (the addition of Gaussian noise to the network weights during training). Weight noise was added once per training sequence, rather than at every timestep. Weight noise tends to ‘simplify’ neural networks, in the sense of reducing the amount of information required to transmit the parameters, which improves generalisation.
In a prior 2011 paper that studies different types of static and adaptive weight noise titled “Practical Variational Inference for Neural Networks,” Graves recommends using early stopping in conjunction with the addition of weight noise with LSTMs.
… in practice early stopping is required to prevent overfitting when training with weight noise.
Tips for Adding Noise During Training
This section provides some tips for adding noise during training with your neural network.
Problem Types for Adding Noise
Noise can be added to training regardless of the type of problem that is being addressed.
It is appropriate to try adding noise to both classification and regression type problems.
The type of noise can be specialized to the types of data used as input to the model, for example, two-dimensional noise in the case of images and signal noise in the case of audio data.
Add Noise to Different Network Types
Adding noise during training is a generic method that can be used regardless of the type of neural network that is being used.
It was a method used primarily with multilayer Perceptrons given their prior dominance, but can be and is used with Convolutional and Recurrent Neural Networks.
Rescale Data First
It is important that the addition of noise has a consistent effect on the model.
This requires that the input data is rescaled so that all variables have the same scale, so that when noise is added to the inputs with a fixed variance, it has the same effect. The also applies to adding noise to weights and gradients as they too are affected by the scale of the inputs.
This can be achieved via standardization or normalization of input variables.
If random noise is added after data scaling, then the variables may need to be rescaled again, perhaps per mini-batch.
Test the Amount of Noise
You cannot know how much noise will benefit your specific model on your training dataset.
Experiment with different amounts, and even different types of noise, in order to discover what works best.
Be systematic and use controlled experiments, perhaps on smaller datasets across a range of values.
Noisy Training Only
Noise is only added during the training of your model.
Be sure that any source of noise is not added during the evaluation of your model, or when your model is used to make predictions on new data.
Further Reading
This section provides more resources on the topic if you are looking to go deeper.
Books
- Section 7.5 Noise Robustness, Deep Learning, 2016.
- Chapter 17 Training with Noisy Inputs, Neural Smithing: Supervised Learning in Feedforward Artificial Neural Networks, 1999.
- Section 9.3, Training with Noise, Neural Networks for Pattern Recognition, 1995.
Papers
- Creating artificial neural networks that generalize, 1991.
- Deep networks for robust visual recognition, 2010.
- Stacked Denoising Autoencoders: Learning Useful Representations in a Deep Network with a Local Denoising Criterion, 2010.
- Analyzing noise in autoencoders and deep networks, 2014.
- The Effects of Adding Noise During Backpropagation Training on a Generalization Performance, 1996.
- Training with Noise is Equivalent to Tikhonov Regularization, 2008.
- Adding Gradient Noise Improves Learning for Very Deep Networks, 2016.
- Noisy Activation Functions, 2016.
Articles
Summary
In this post, you discovered that adding noise to a neural network during training can improve the robustness of the network resulting in better generalization and faster learning.
Specifically, you learned:
- Small datasets can make learning challenging for neural nets and the examples can be memorized.
- Adding noise during training can make the training process more robust and reduce generalization error.
- Noise is traditionally added to the inputs, but can also be added to weights, gradients, and even activation functions.
Do you have any questions?
Ask your questions in the comments below and I will do my best to answer.
The post Train Neural Networks With Noise to Reduce Overfitting appeared first on Machine Learning Mastery.