Author: Jason Brownlee
It is critical that any data preparation performed on a training dataset is also performed on a new dataset in the future.
This may include a test dataset when evaluating a model or new data from the domain when using a model to make predictions.
Typically, the model fit on the training dataset is saved for later use. The correct solution to preparing new data for the model in the future is to also save any data preparation objects, like data scaling methods, to file along with the model.
In this tutorial, you will discover how to save a model and data preparation object to file for later use.
After completing this tutorial, you will know:
- The challenge of correctly preparing test data and new data for a machine learning model.
- The solution of saving the model and data preparation objects to file for later use.
- How to save and later load and use a machine learning model and data preparation model on new data.
Let’s get started.
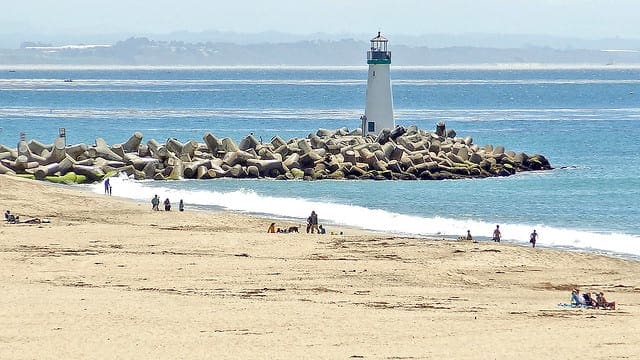
How to Save and Load Models and Data Preparation in Scikit-Learn for Later Use
Photo by Dennis Jarvis, some rights reserved.
Tutorial Overview
This tutorial is divided into three parts; they are:
- Challenging of Preparing New Data for a Model
- Save Data Preparation Objects
- How to Save and Later Use a Data Preparation Object
Challenging of Preparing New Data for a Model
Each input variable in a dataset may have different units.
For example, one variable may be in inches, another in miles, another in days, and so on.
As such, it is often important to scale data prior to fitting a model.
This is particularly important for models that use a weighted sum of the input or distance measures like logistic regression, neural networks, and k-nearest neighbors. This is because variables with larger values or ranges may dominate or wash out the effects of variables with smaller values or ranges.
Scaling techniques, such as normalization or standardization, have the effect of transforming the distribution of each input variable to be the same, such as the same minimum and maximum in the case of normalization or the same mean and standard deviation in the case of standardization.
A scaling technique must be fit, which just means it needs to calculate coefficients from data, such as the observed min and max, or the observed mean and standard deviation. These values can also be set by domain experts.
The best practice when using scaling techniques for evaluating models is to fit them on the training dataset, then apply them to the training and test datasets.
Or, when working with a final model, to fit the scaling method on the training dataset and apply the transform to the training dataset and any new dataset in the future.
It is critical that any data preparation or transformation applied to the training dataset is also applied to the test or other dataset in the future.
This is straightforward when all of the data and the model are in memory.
This is challenging when a model is saved and used later.
What is the best practice to scale data when saving a fit model for later use, such as a final model?
The Solution: Save Data Preparation Objects
The solution is to save the data preparation object to file along with the model.
For example, it is common to use the pickle framework (built-in to Python) for saving machine learning models for later use, such as saving a final model.
This same framework can be used to save the object that was used for data preparation.
Later, the model and the data preparation object can be loaded and used.
It is convenient to save the entire objects to file, such as the model object and the data preparation object. Nevertheless, experts may prefer to save just the model parameters to file, then load them later and set them into a new model object. This approach can also be used with the coefficients used for scaling the data, such as the min and max values for each variable, or the mean and standard deviation for each variable.
The choice of which approach is appropriate for your project is up to you, but I recommend saving the model and data preparation object (or objects) to file directly for later use.
To make the idea of saving the object and data transform object to file concrete, let’s look at a worked example.
How to Save and Later Use a Data Preparation Object
In this section, we will demonstrate preparing a dataset, fitting a model on the dataset, saving the model and data transform object to file, and later loading the model and transform and using them on new data.
1. Define a Dataset
First, we need a dataset.
We will use a test dataset from the scikit-learn dataset, specifically a binary classification problem with two input variables created randomly via the make_blobs() function.
The example below creates a test dataset with 100 examples, two input features, and two class labels (0 and 1). The dataset is then split into training and test sets and the min and max values of each variable are then reported.
Importantly, the random_state is set when creating the dataset and when splitting the data so that the same dataset is created and the same split of data is performed each time that the code is run.
# example of creating a test dataset and splitting it into train and test sets from sklearn.datasets.samples_generator import make_blobs from sklearn.model_selection import train_test_split # prepare dataset X, y = make_blobs(n_samples=100, centers=2, n_features=2, random_state=1) # split data into train and test sets X_train, X_test, y_train, y_test = train_test_split(X, y, test_size=0.33, random_state=1) # summarize dataset for i in range(X.shape[1]): print('Train', i, X_train[i].min(), X_train[i].max()) print('Test', i, X_test[i].min(), X_test[i].max())
Running the example reports the min and max values for each variable in both the train and test datasets.
We can see that each variable has a different scale, and that the scales differ between the train and test datasets. This is a realistic scenario that we may encounter with a real dataset.
Train 0 -8.958887901793688 -1.766368900388947 Test 0 -0.5279305184970926 5.92630668526536 Train 1 -1.9657639185768914 5.234464511450407 Test 1 -2.351220657673829 4.0097363419871845
2. Scale the Dataset
Next, we can scale the dataset.
We will use the MinMaxScaler to scale each input variable to the range [0, 1]. The best practice use of this scaler is to fit it on the training dataset and then apply the transform to the training dataset, and other datasets: in this case, the test dataset.
The complete example of scaling the data and summarizing the effects is listed below.
# example of scaling the dataset from sklearn.datasets.samples_generator import make_blobs from sklearn.model_selection import train_test_split from sklearn.preprocessing import MinMaxScaler # prepare dataset X, y = make_blobs(n_samples=100, centers=2, n_features=2, random_state=1) # split data into train and test sets X_train, X_test, y_train, y_test = train_test_split(X, y, test_size=0.33, random_state=1) # define scaler scaler = MinMaxScaler() # fit scaler on the training dataset scaler.fit(X_train) # transform both datasets X_train_scaled = scaler.transform(X_train) X_test_scaled = scaler.transform(X_test) # summarize dataset for i in range(X.shape[1]): print('Train', i, X_train_scaled[i].min(), X_train_scaled[i].max()) print('Test', i, X_test_scaled[i].min(), X_test_scaled[i].max())
Running the example prints the effect of the scaled data showing the min and max values for each variable in the train and test datasets.
We can see that all variables in both datasets now have values in the desired range of 0 to 1.
Train 0 0.23395851599080797 0.35842125076406284 Test 0 0.9148787986264039 0.9549870948672079 Train 1 0.7987532056890075 0.901334837494266 Test 1 0.7676220660334238 0.8063573506527247
3. Save Model and Data Scaler
Next, we can fit a model on the training dataset and save both the model and the scaler object to file.
We will use a LogisticRegression model because the problem is a simple binary classification task.
The training dataset is scaled as before, and in this case, we will assume the test dataset is currently not available. Once scaled, the dataset is used to fit a logistic regression model.
We will use the pickle framework to save the LogisticRegression model to one file, and the MinMaxScaler to another file.
The complete example is listed below.
# example of fitting a model on the scaled dataset from sklearn.datasets.samples_generator import make_blobs from sklearn.model_selection import train_test_split from sklearn.preprocessing import MinMaxScaler from sklearn.linear_model import LogisticRegression from pickle import dump # prepare dataset X, y = make_blobs(n_samples=100, centers=2, n_features=2, random_state=1) # split data into train and test sets X_train, _, y_train, _ = train_test_split(X, y, test_size=0.33, random_state=1) # define scaler scaler = MinMaxScaler() # fit scaler on the training dataset scaler.fit(X_train) # transform the training dataset X_train_scaled = scaler.transform(X_train) # define model model = LogisticRegression(solver='lbfgs') model.fit(X_train_scaled, y_train) # save the model dump(model, open('model.pkl', 'wb')) # save the scaler dump(scaler, open('scaler.pkl', 'wb'))
Running the example scales the data, fits the model, and saves the model and scaler to files using pickle.
You should have two files in your current working directory:
- model.pkl
- scaler.pkl
4. Load Model and Data Scaler
Finally, we can load the model and the scaler object and make use of them.
In this case, we will assume that the training dataset is not available, and that only new data or the test dataset is available.
We will load the model and the scaler, then use the scaler to prepare the new data and use the model to make predictions. Because it is a test dataset, we have the expected target values, so we will compare the predictions to the expected target values and calculate the accuracy of the model.
The complete example is listed below.
# load model and scaler and make predictions on new data from sklearn.datasets.samples_generator import make_blobs from sklearn.model_selection import train_test_split from sklearn.metrics import accuracy_score from pickle import load # prepare dataset X, y = make_blobs(n_samples=100, centers=2, n_features=2, random_state=1) # split data into train and test sets _, X_test, _, y_test = train_test_split(X, y, test_size=0.33, random_state=1) # load the model model = load(open('model.pkl', 'rb')) # save the scaler scaler = load(open('scaler.pkl', 'rb')) # transform the test dataset X_test_scaled = scaler.transform(X_test) # make predictions on the test set yhat = model.predict(X_test_scaled) # evaluate accuracy acc = accuracy_score(y_test, yhat) print('Test Accuracy:', acc)
Running the example loads the model and scaler, then uses the scaler to prepare the test dataset correctly for the model, meeting the expectations of the model when it was trained.
The model then makes a prediction for the examples in the test set and the classification accuracy is calculated. In this case, the model achieved 100% accuracy on the test set because the test problem is trivial.
Test Accuracy: 1.0
This provides a template that you can use to save both your model and scaler object (or objects) to file on your own projects.
Further Reading
This section provides more resources on the topic if you are looking to go deeper.
Posts
- Save and Load Machine Learning Models in Python with scikit-learn
- How to Train a Final Machine Learning Model
APIs
- sklearn.datasets.make_blobs API.
- sklearn.model_selection.train_test_split API.
- sklearn.preprocessing.MinMaxScaler API.
- sklearn.metrics.accuracy_score API.
- sklearn.linear_model.LogisticRegression API.
- pickle API.
Summary
In this tutorial, you discovered how to save a model and data preparation object to file for later use.
Specifically, you learned:
- The challenge of correctly preparing test data and new data for a machine learning model.
- The solution of saving the model and data preparation objects to file for later use.
- How to save and later load and use a machine learning model and data preparation model on new data.
Do you have any questions?
Ask your questions in the comments below and I will do my best to answer.
The post How to Save and Reuse Data Preparation Objects in Scikit-Learn appeared first on Machine Learning Mastery.