Author: Jason Brownlee
Classification predictive modeling involves predicting a class label for examples, although some problems require the prediction of a probability of class membership.
For these problems, the crisp class labels are not required, and instead, the likelihood that each example belonging to each class is required and later interpreted. As such, small relative probabilities can carry a lot of meaning and specialized metrics are required to quantify the predicted probabilities.
In this tutorial, you will discover metrics for evaluating probabilistic predictions for imbalanced classification.
After completing this tutorial, you will know:
- Probability predictions are required for some classification predictive modeling problems.
- Log loss quantifies the average difference between predicted and expected probability distributions.
- Brier score quantifies the average difference between predicted and expected probabilities.
Let’s get started.
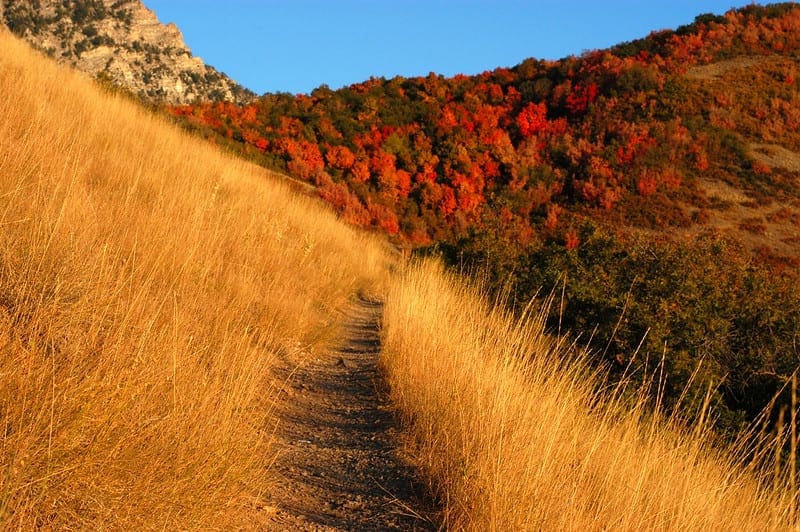
A Gentle Introduction to Probability Metrics for Imbalanced Classification
Photo by a4gpa, some rights reserved.
Tutorial Overview
This tutorial is divided into three parts; they are:
- Probability Metrics
- Log Loss for Imbalanced Classification
- Brier Score for Imbalanced Classification
Probability Metrics
Classification predictive modeling involves predicting a class label for an example.
On some problems, a crisp class label is not required, and instead a probability of class membership is preferred. The probability summarizes the likelihood (or uncertainty) of an example belonging to each class label. Probabilities are more nuanced and can be interpreted by a human operator or a system in decision making.
Probability metrics are those specifically designed to quantify the skill of a classifier model using the predicted probabilities instead of crisp class labels. They are typically scores that provide a single value that can be used to compare different models based on how well the predicted probabilities match the expected class probabilities.
In practice, a dataset will not have target probabilities. Instead, it will have class labels.
For example, a two-class (binary) classification problem will have the class labels 0 for the negative case and 1 for the positive case. When an example has the class label 0, then the probability of the class labels 0 and 1 will be 1 and 0 respectively. When an example has the class label 1, then the probability of class labels 0 and 1 will be 0 and 1 respectively.
- Example with Class=0: P(class=0) = 1, P(class=1) = 0
- Example with Class=1: P(class=0) = 0, P(class=1) = 1
We can see how this would scale to three classes or more; for example:
- Example with Class=0: P(class=0) = 1, P(class=1) = 0, P(class=2) = 0
- Example with Class=1: P(class=0) = 0, P(class=1) = 1, P(class=2) = 0
- Example with Class=2: P(class=0) = 0, P(class=1) = 0, P(class=2) = 1
In the case of binary classification problems, this representation can be simplified to just focus on the positive class.
That is, we only require the probability of an example belonging to class 1 to represent the probabilities for binary classification (the so-called Bernoulli distribution); for example:
- Example with Class=0: P(class=1) = 0
- Example with Class=1: P(class=1) = 1
Probability metrics will summarize how well the predicted distribution of class membership matches the known class probability distribution.
This focus on predicted probabilities may mean that the crisp class labels predicted by a model are ignored. This focus may mean that a model that predicts probabilities may appear to have terrible performance when evaluated according to its crisp class labels, such as using accuracy or a similar score. This is because although the predicted probabilities may show skill, they must be interpreted with an appropriate threshold prior to being converted into crisp class labels.
Additionally, the focus on predicted probabilities may also require that the probabilities predicted by some nonlinear models to be calibrated prior to being used or evaluated. Some models will learn calibrated probabilities as part of the training process (e.g. logistic regression), but many will not and will require calibration (e.g. support vector machines, decision trees, and neural networks).
A given probability metric is typically calculated for each example, then averaged across all examples in the training dataset.
There are two popular metrics for evaluating predicted probabilities; they are:
- Log Loss
- Brier Score
Let’s take a closer look at each in turn.
Log Loss for Imbalanced Classification
Logarithmic loss or log loss for short is a loss function known for training the logistic regression classification algorithm.
The log loss function calculates the negative log likelihood for probability predictions made by the binary classification model. Most notably, this is logistic regression, but this function can be used by other models, such as neural networks, and is known by other names, such as cross-entropy.
Generally, the log loss can be calculated using the expected probabilities for each class and the natural logarithm of the predicted probabilities for each class; for example:
- LogLoss = -(P(class=0) * log(P(class=0)) + (P(class=1)) * log(P(class=1)))
The best possible log loss is 0.0, and values are positive to infinite for progressively worse scores.
If you are just predicting the probability for the positive class, then the log loss function can be calculated for one binary classification prediction (yhat) compared to the expected probability (y) as follows:
- LogLoss = -((1 – y) * log(1 – yhat) + y * log(yhat))
For example, if the expected probability was 1.0 and the model predicted 0.8, the log loss would be:
- LogLoss = -((1 – y) * log(1 – yhat) + y * log(yhat))
- LogLoss = -((1 – 1.0) * log(1 – 0.8) + 1.0 * log(0.8))
- LogLoss = -(-0.0 + -0.223)
- LogLoss = 0.223
This calculation can be scaled up for multiple classes by adding additional terms; for example:
- LogLoss = -( sum c in C y_c * log(yhat_c))
This generalization is also known as cross-entropy and calculates the number of bits (if log base-2 is used) or nats (if log base-e is used) by which two probability distributions differ.
Specifically, it builds upon the idea of entropy from information theory and calculates the average number of bits required to represent or transmit an event from one distribution compared to the other distribution.
… the cross entropy is the average number of bits needed to encode data coming from a source with distribution p when we use model q …
— Page 57, Machine Learning: A Probabilistic Perspective, 2012.
The intuition for this definition comes if we consider a target or underlying probability distribution P and an approximation of the target distribution Q, then the cross-entropy of Q from P is the number of additional bits to represent an event using Q instead of P.
We will stick with log loss for now, as it is the term most commonly used when using this calculation as an evaluation metric for classifier models.
When calculating the log loss for a set of predictions compared to a set of expected probabilities in a test dataset, the average of the log loss across all samples is calculated and reported; for example:
- AverageLogLoss = 1/N * sum i in N -((1 – y) * log(1 – yhat) + y * log(yhat))
The average log loss for a set of predictions on a training dataset is often simply referred to as the log loss.
We can demonstrate calculating log loss with a worked example.
First, let’s define a synthetic binary classification dataset. We will use the make_classification() function to create 1,000 examples, with 99%/1% split for the two classes. The complete example of creating and summarizing the dataset is listed below.
# create an imbalanced dataset from numpy import unique from sklearn.datasets import make_classification # generate 2 class dataset X, y = make_classification(n_samples=1000, n_classes=2, weights=[0.99], flip_y=0, random_state=1) # summarize dataset classes = unique(y) total = len(y) for c in classes: n_examples = len(y[y==c]) percent = n_examples / total * 100 print('> Class=%d : %d/%d (%.1f%%)' % (c, n_examples, total, percent))
Running the example creates the dataset and reports the distribution of examples in each class.
> Class=0 : 990/1000 (99.0%) > Class=1 : 10/1000 (1.0%)
Next, we will develop an intuition for naive predictions of probabilities.
A naive prediction strategy would be to predict certainty for the majority class, or P(class=0) = 1. An alternative strategy would be to predict the minority class, or P(class=1) = 1.
Log loss can be calculated using the log_loss() scikit-learn function. It takes the probability for each class as input and returns the average log loss. Specifically, each example must have a prediction with one probability per class, meaning a prediction for one example for a binary classification problem must have a probability for class 0 and class 1.
Therefore, predicting certain probabilities for class 0 for all examples would be implemented as follows:
... # no skill prediction 0 probabilities = [[1, 0] for _ in range(len(testy))] avg_logloss = log_loss(testy, probabilities) print('P(class0=1): Log Loss=%.3f' % (avg_logloss))
We can do the same thing for P(class1)=1.
These two strategies are expected to perform terribly.
A better naive strategy would be to predict the class distribution for each example. For example, because our dataset has a 99%/1% class distribution for the majority and minority classes, this distribution can be “predicted” for each example to give a baseline for probability predictions.
... # baseline probabilities probabilities = [[0.99, 0.01] for _ in range(len(testy))] avg_logloss = log_loss(testy, probabilities) print('Baseline: Log Loss=%.3f' % (avg_logloss))
Finally, we can also calculate the log loss for perfectly predicted probabilities by taking the target values for the test set as predictions.
... # perfect probabilities avg_logloss = log_loss(testy, testy) print('Perfect: Log Loss=%.3f' % (avg_logloss))
Tying this all together, the complete example is listed below.
# log loss for naive probability predictions. from numpy import mean from sklearn.datasets import make_classification from sklearn.model_selection import train_test_split from sklearn.metrics import log_loss # generate 2 class dataset X, y = make_classification(n_samples=1000, n_classes=2, weights=[0.99], flip_y=0, random_state=1) # split into train/test sets with same class ratio trainX, testX, trainy, testy = train_test_split(X, y, test_size=0.5, random_state=2, stratify=y) # no skill prediction 0 probabilities = [[1, 0] for _ in range(len(testy))] avg_logloss = log_loss(testy, probabilities) print('P(class0=1): Log Loss=%.3f' % (avg_logloss)) # no skill prediction 1 probabilities = [[0, 1] for _ in range(len(testy))] avg_logloss = log_loss(testy, probabilities) print('P(class1=1): Log Loss=%.3f' % (avg_logloss)) # baseline probabilities probabilities = [[0.99, 0.01] for _ in range(len(testy))] avg_logloss = log_loss(testy, probabilities) print('Baseline: Log Loss=%.3f' % (avg_logloss)) # perfect probabilities avg_logloss = log_loss(testy, testy) print('Perfect: Log Loss=%.3f' % (avg_logloss))
Running the example reports the log loss for each naive strategy.
As expected, predicting certainty for each class label is punished with large log loss scores, with the case of being certain for the minority class in all cases resulting in a much larger score.
We can see that predicting the distribution of examples in the dataset as the baseline results in a better score than either of the other naive measures. This baseline represents the no skill classifier and log loss scores below this strategy represent a model that has some skill.
Finally, we can see that a log loss for perfectly predicted probabilities is 0.0, indicating no difference between actual and predicted probability distributions.
P(class0=1): Log Loss=0.345 P(class1=1): Log Loss=34.193 Baseline: Log Loss=0.056 Perfect: Log Loss=0.000
Now that we are familiar with log loss, let’s take a look at the Brier score.
Brier Score for Imbalanced Classification
The Brier score, named for Glenn Brier, calculates the mean squared error between predicted probabilities and the expected values.
The score summarizes the magnitude of the error in the probability forecasts and is designed for binary classification problems. It is focused on evaluating the probabilities for the positive class. Nevertheless, it can be adapted for problems with multiple classes.
As such, it is an appropriate probabilistic metric for imbalanced classification problems.
The evaluation of probabilistic scores is generally performed by means of the Brier Score. The basic idea is to compute the mean squared error (MSE) between predicted probability scores and the true class indicator, where the positive class is coded as 1, and negative class 0.
— Page 57, Learning from Imbalanced Data Sets, 2018.
The error score is always between 0.0 and 1.0, where a model with perfect skill has a score of 0.0.
The Brier score can be calculated for positive predicted probabilities (yhat) compared to the expected probabilities (y) as follows:
- BrierScore = 1/N * Sum i to N (yhat_i – y_i)^2
For example, if a predicted positive class probability is 0.8 and the expected probability is 1.0, then the Brier score is calculated as:
- BrierScore = (yhat_i – y_i)^2
- BrierScore = (0.8 – 1.0)^2
- BrierScore = 0.04
We can demonstrate calculating Brier score with a worked example using the same dataset and naive predictive models as were used in the previous section.
The Brier score can be calculated using the brier_score_loss() scikit-learn function. It takes the probabilities for the positive class only, and returns an average score.
As in the previous section, we can evaluate naive strategies of predicting the certainty for each class label. In this case, as the score only considered the probability for the positive class, this will involve predicting 0.0 for P(class=1)=0 and 1.0 for P(class=1)=1. For example:
... # no skill prediction 0 probabilities = [0.0 for _ in range(len(testy))] avg_brier = brier_score_loss(testy, probabilities) print('P(class1=0): Brier Score=%.4f' % (avg_brier)) # no skill prediction 1 probabilities = [1.0 for _ in range(len(testy))] avg_brier = brier_score_loss(testy, probabilities) print('P(class1=1): Brier Score=%.4f' % (avg_brier))
We can also test the no skill classifier that predicts the ratio of positive examples in the dataset, which in this case is 1 percent or 0.01.
... # baseline probabilities probabilities = [0.01 for _ in range(len(testy))] avg_brier = brier_score_loss(testy, probabilities) print('Baseline: Brier Score=%.4f' % (avg_brier))
Finally, we can also confirm the Brier score for perfectly predicted probabilities.
... # perfect probabilities avg_brier = brier_score_loss(testy, testy) print('Perfect: Brier Score=%.4f' % (avg_brier))
Tying this together, the complete example is listed below.
# brier score for naive probability predictions. from numpy import mean from sklearn.datasets import make_classification from sklearn.model_selection import train_test_split from sklearn.metrics import brier_score_loss # generate 2 class dataset X, y = make_classification(n_samples=1000, n_classes=2, weights=[0.99], flip_y=0, random_state=1) # split into train/test sets with same class ratio trainX, testX, trainy, testy = train_test_split(X, y, test_size=0.5, random_state=2, stratify=y) # no skill prediction 0 probabilities = [0.0 for _ in range(len(testy))] avg_brier = brier_score_loss(testy, probabilities) print('P(class1=0): Brier Score=%.4f' % (avg_brier)) # no skill prediction 1 probabilities = [1.0 for _ in range(len(testy))] avg_brier = brier_score_loss(testy, probabilities) print('P(class1=1): Brier Score=%.4f' % (avg_brier)) # baseline probabilities probabilities = [0.01 for _ in range(len(testy))] avg_brier = brier_score_loss(testy, probabilities) print('Baseline: Brier Score=%.4f' % (avg_brier)) # perfect probabilities avg_brier = brier_score_loss(testy, testy) print('Perfect: Brier Score=%.4f' % (avg_brier))
Running the example, we can see the scores for the naive models and the baseline no skill classifier.
As we might expect, we can see that predicting a 0.0 for all examples results in a low score, as the mean squared error between all 0.0 predictions and mostly 0 classes in the test set results in a small value. Conversely, the error between 1.0 predictions and mostly 0 class values results in a larger error score.
Importantly, we can see that the default no skill classifier results in a lower score than predicting all 0.0 values. Again, this represents the baseline score, below which models will demonstrate skill.
P(class1=0): Brier Score=0.0100 P(class1=1): Brier Score=0.9900 Baseline: Brier Score=0.0099 Perfect: Brier Score=0.0000
The Brier scores can become very small and the focus will be on fractions well below the decimal point. For example, the difference in the above example between Baseline and Perfect scores is slight at four decimal places.
A common practice is to transform the score using a reference score, such as the no skill classifier. This is called a Brier Skill Score, or BSS, and is calculated as follows:
- BrierSkillScore = 1 – (BrierScore / BrierScore_ref)
We can see that if the reference score was evaluated, it would result in a BSS of 0.0. This represents a no skill prediction. Values below this will be negative and represent worse than no skill. Values above 0.0 represent skillful predictions with a perfect prediction value of 1.0.
We can demonstrate this by developing a function to calculate the Brier skill score listed below.
# calculate the brier skill score def brier_skill_score(y, yhat, brier_ref): # calculate the brier score bs = brier_score_loss(y, yhat) # calculate skill score return 1.0 - (bs / brier_ref)
We can then calculate the BSS for each of the naive forecasts, as well as for a perfect prediction.
The complete example is listed below.
# brier skill score for naive probability predictions. from numpy import mean from sklearn.datasets import make_classification from sklearn.model_selection import train_test_split from sklearn.metrics import brier_score_loss # calculate the brier skill score def brier_skill_score(y, yhat, brier_ref): # calculate the brier score bs = brier_score_loss(y, yhat) # calculate skill score return 1.0 - (bs / brier_ref) # generate 2 class dataset X, y = make_classification(n_samples=1000, n_classes=2, weights=[0.99], flip_y=0, random_state=1) # split into train/test sets with same class ratio trainX, testX, trainy, testy = train_test_split(X, y, test_size=0.5, random_state=2, stratify=y) # calculate reference probabilities = [0.01 for _ in range(len(testy))] brier_ref = brier_score_loss(testy, probabilities) print('Reference: Brier Score=%.4f' % (brier_ref)) # no skill prediction 0 probabilities = [0.0 for _ in range(len(testy))] bss = brier_skill_score(testy, probabilities, brier_ref) print('P(class1=0): BSS=%.4f' % (bss)) # no skill prediction 1 probabilities = [1.0 for _ in range(len(testy))] bss = brier_skill_score(testy, probabilities, brier_ref) print('P(class1=1): BSS=%.4f' % (bss)) # baseline probabilities probabilities = [0.01 for _ in range(len(testy))] bss = brier_skill_score(testy, probabilities, brier_ref) print('Baseline: BSS=%.4f' % (bss)) # perfect probabilities bss = brier_skill_score(testy, testy, brier_ref) print('Perfect: BSS=%.4f' % (bss))
Running the example first calculates the reference Brier score used in the BSS calculation.
We can then see that predicting certainty scores for each class results in a negative BSS score, indicating that they are worse than no skill. Finally, we can see that evaluating the reference forecast itself results in 0.0, indicating no skill and evaluating the true values as predictions results in a perfect score of 1.0.
As such, the Brier Skill Score is a best practice for evaluating probability predictions and is widely used where probability classification prediction are evaluated routinely, such as in weather forecasts (e.g. rain or not).
Reference: Brier Score=0.0099 P(class1=0): BSS=-0.0101 P(class1=1): BSS=-99.0000 Baseline: BSS=0.0000 Perfect: BSS=1.0000
Further Reading
This section provides more resources on the topic if you are looking to go deeper.
Tutorials
- A Gentle Introduction to Probability Scoring Methods in Python
- A Gentle Introduction to Cross-Entropy for Machine Learning
- A Gentle Introduction to Logistic Regression With Maximum Likelihood Estimation
Books
- Chapter 8 Assessment Metrics For Imbalanced Learning, Imbalanced Learning: Foundations, Algorithms, and Applications, 2013.
- Chapter 3 Performance Measures, Learning from Imbalanced Data Sets, 2018.
API
- sklearn.datasets.make_classification API.
- sklearn.metrics.log_loss API.
- sklearn.metrics.brier_score_loss API.
Articles
- Brier score, Wikipedia.
- Cross entropy, Wikipedia.
- Joint Working Group on Forecast Verification Research
Summary
In this tutorial, you discovered metrics for evaluating probabilistic predictions for imbalanced classification.
Specifically, you learned:
- Probability predictions are required for some classification predictive modeling problems.
- Log loss quantifies the average difference between predicted and expected probability distributions.
- Brier score quantifies the average difference between predicted and expected probabilities.
Do you have any questions?
Ask your questions in the comments below and I will do my best to answer.
The post A Gentle Introduction to Probability Metrics for Imbalanced Classification appeared first on Machine Learning Mastery.