Author: Jason Brownlee
Data cleaning is a critically important step in any machine learning project.
In tabular data, there are many different statistical analysis and data visualization techniques you can use to explore your data in order to identify data cleaning operations you may want to perform.
Before jumping to the sophisticated methods, there are some very basic data cleaning operations that you probably should perform on every single machine learning project. These are so basic that they are often overlooked by seasoned machine learning practitioners, yet are so critical that if skipped, models may break or report overly optimistic performance results.
In this tutorial, you will discover basic data cleaning you should always perform on your dataset.
After completing this tutorial, you will know:
- How to identify and remove column variables that only have a single value.
- How to identify and consider column variables with very few unique values.
- How to identify and remove rows that contain duplicate observations.
Let’s get started.
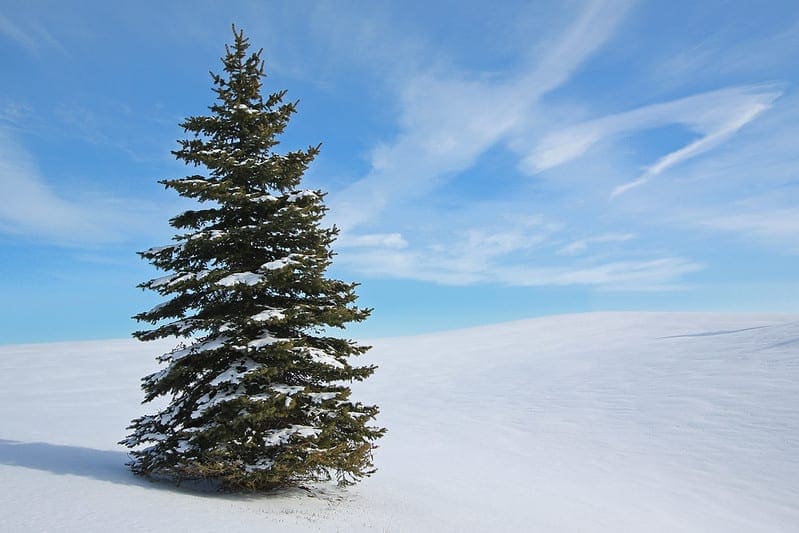
Basic Data Cleaning You Must Perform in Machine Learning
Photo by Allen McGregor, some rights reserved.
Tutorial Overview
This tutorial is divided into five parts; they are:
- Identify Columns That Contain a Single Value
- Delete Columns That Contain a Single Value
- Consider Columns That Have Very Few Values
- Identify Rows that Contain Duplicate Data
- Delete Rows that Contain Duplicate Data
Identify Columns That Contain a Single Value
Columns that have a single observation or value are probably useless for modeling.
Here, a single value means that each row for that column has the same value. For example, the column X1 has the value 1.0 for all rows in the dataset:
X1 1.0 1.0 1.0 1.0 1.0 ...
Columns that have a single value for all rows do not contain any information for modeling.
Depending on the choice of data preparation and modeling algorithms, variables with a single value can also cause errors or unexpected results.
You can detect rows that have this property using the unique() NumPy function that will report the number of unique values in each column.
The example below loads the oil-spill classification dataset that contains 50 variables and summarizes the number of unique values for each column.
# summarize the number of unique values for each column using numpy from urllib.request import urlopen from numpy import loadtxt from numpy import unique # define the location of the dataset path = 'https://raw.githubusercontent.com/jbrownlee/Datasets/master/oil-spill.csv' # load the dataset data = loadtxt(urlopen(path), delimiter=',') # summarize the number of unique values in each column for i in range(data.shape[1]): print(i, len(unique(data[:, i])))
Running the example loads the dataset directly from the URL and prints the number of unique values for each column.
We can see that column index 22 only has a single value and should be removed.
0 238 1 297 2 927 3 933 4 179 5 375 6 820 7 618 8 561 9 57 10 577 11 59 12 73 13 107 14 53 15 91 16 893 17 810 18 170 19 53 20 68 21 9 22 1 23 92 24 9 25 8 26 9 27 308 28 447 29 392 30 107 31 42 32 4 33 45 34 141 35 110 36 3 37 758 38 9 39 9 40 388 41 220 42 644 43 649 44 499 45 2 46 937 47 169 48 286 49 2
A simpler approach is to use the nunique() Pandas function that does the hard work for you.
Below is the same example using the Pandas function.
# summarize the number of unique values for each column using numpy from pandas import read_csv # define the location of the dataset path = 'https://raw.githubusercontent.com/jbrownlee/Datasets/master/oil-spill.csv' # load the dataset df = read_csv(path, header=None) # summarize the number of unique values in each column print(df.nunique())
Running the example, we get the same result, the column index, and the number of unique values for each column.
0 238 1 297 2 927 3 933 4 179 5 375 6 820 7 618 8 561 9 57 10 577 11 59 12 73 13 107 14 53 15 91 16 893 17 810 18 170 19 53 20 68 21 9 22 1 23 92 24 9 25 8 26 9 27 308 28 447 29 392 30 107 31 42 32 4 33 45 34 141 35 110 36 3 37 758 38 9 39 9 40 388 41 220 42 644 43 649 44 499 45 2 46 937 47 169 48 286 49 2 dtype: int64
Delete Columns That Contain a Single Value
Variables or columns that have a single value should probably be removed from your dataset
Columns are relatively easy to remove from a NumPy array or Pandas DataFrame.
One approach is to record all columns that have a single unique value, then delete them from the Pandas DataFrame by calling the drop() function.
The complete example is listed below.
# delete columns with a single unique value from pandas import read_csv # define the location of the dataset path = 'https://raw.githubusercontent.com/jbrownlee/Datasets/master/oil-spill.csv' # load the dataset df = read_csv(path, header=None) print(df.shape) # get number of unique values for each column counts = df.nunique() # record columns to delete to_del = [i for i,v in enumerate(counts) if v == 1] print(to_del) # drop useless columns df.drop(to_del, axis=1, inplace=True) print(df.shape)
Running the example first loads the dataset and reports the number of rows and columns.
The number of unique values for each column is calculated, and those columns that have a single unique value are identified. In this case, column index 22.
The identified columns are then removed from the DataFrame, and the number of rows and columns in the DataFrame are reported to confirm the change.
(937, 50) [22] (937, 49)
Consider Columns That Have Very Few Values
In the previous section, we saw that some columns in the example dataset had very few unique values.
For example, there were columns that only had 2, 4, and 9 unique values. This might make sense for ordinal or categorical variables. In this case, the dataset only contains numerical variables. As such, only having 2, 4, or 9 unique numerical values in a column might be surprising.
These columns may or may not contribute to the skill of a model.
Depending on the choice of data preparation and modeling algorithms, variables with very few numerical values can also cause errors or unexpected results. For example, I have seen them cause errors when using power transforms for data preparation and when fitting linear models that assume a “sensible” data probability distribution.
To help highlight columns of this type, you can calculate the number of unique values for each variable as a percentage of the total number of rows in the dataset.
Let’s do this manually using NumPy. The complete example is listed below.
# summarize the percentage of unique values for each column using numpy from urllib.request import urlopen from numpy import loadtxt from numpy import unique # define the location of the dataset path = 'https://raw.githubusercontent.com/jbrownlee/Datasets/master/oil-spill.csv' # load the dataset data = loadtxt(urlopen(path), delimiter=',') # summarize the number of unique values in each column for i in range(data.shape[1]): num = len(unique(data[:, i])) percentage = float(num) / data.shape[0] * 100 print('%d, %d, %.1f%%' % (i, num, percentage))
Running the example reports the column index and the number of unique values for each column, followed by the percentage of unique values out of all rows in the dataset.
Here, we can see that some columns have a very low percentage of unique values, such as below 1 percent.
0, 238, 25.4% 1, 297, 31.7% 2, 927, 98.9% 3, 933, 99.6% 4, 179, 19.1% 5, 375, 40.0% 6, 820, 87.5% 7, 618, 66.0% 8, 561, 59.9% 9, 57, 6.1% 10, 577, 61.6% 11, 59, 6.3% 12, 73, 7.8% 13, 107, 11.4% 14, 53, 5.7% 15, 91, 9.7% 16, 893, 95.3% 17, 810, 86.4% 18, 170, 18.1% 19, 53, 5.7% 20, 68, 7.3% 21, 9, 1.0% 22, 1, 0.1% 23, 92, 9.8% 24, 9, 1.0% 25, 8, 0.9% 26, 9, 1.0% 27, 308, 32.9% 28, 447, 47.7% 29, 392, 41.8% 30, 107, 11.4% 31, 42, 4.5% 32, 4, 0.4% 33, 45, 4.8% 34, 141, 15.0% 35, 110, 11.7% 36, 3, 0.3% 37, 758, 80.9% 38, 9, 1.0% 39, 9, 1.0% 40, 388, 41.4% 41, 220, 23.5% 42, 644, 68.7% 43, 649, 69.3% 44, 499, 53.3% 45, 2, 0.2% 46, 937, 100.0% 47, 169, 18.0% 48, 286, 30.5% 49, 2, 0.2%
We can update the example to only summarize those variables that have unique values that are less than 1 percent of the number of rows.
# summarize the percentage of unique values for each column using numpy from urllib.request import urlopen from numpy import loadtxt from numpy import unique # define the location of the dataset path = 'https://raw.githubusercontent.com/jbrownlee/Datasets/master/oil-spill.csv' # load the dataset data = loadtxt(urlopen(path), delimiter=',') # summarize the number of unique values in each column for i in range(data.shape[1]): num = len(unique(data[:, i])) percentage = float(num) / data.shape[0] * 100 if percentage < 1: print('%d, %d, %.1f%%' % (i, num, percentage))
Running the example, we can see that 11 of the 50 variables have numerical variables that have unique values that are less than 1 percent of the number of rows.
This does not mean that these rows and columns should be deleted, but they require further attention.
For example:
- Perhaps the unique values can be encoded as ordinal values?
- Perhaps the unique values can be encoded as categorical values?
- Perhaps compare model skill with each variable removed from the dataset?
21, 9, 1.0% 22, 1, 0.1% 24, 9, 1.0% 25, 8, 0.9% 26, 9, 1.0% 32, 4, 0.4% 36, 3, 0.3% 38, 9, 1.0% 39, 9, 1.0% 45, 2, 0.2% 49, 2, 0.2%
For example, if we wanted to delete all 11 columns with unique values less than 1 percent of rows; the example below demonstrates this.
# delete columns where number of unique values is less than 1% of the rows from pandas import read_csv # define the location of the dataset path = 'https://raw.githubusercontent.com/jbrownlee/Datasets/master/oil-spill.csv' # load the dataset df = read_csv(path, header=None) print(df.shape) # get number of unique values for each column counts = df.nunique() # record columns to delete to_del = [i for i,v in enumerate(counts) if (float(v)/df.shape[0]*100) < 1] print(to_del) # drop useless columns df.drop(to_del, axis=1, inplace=True) print(df.shape)
Running the example first loads the dataset and reports the number of rows and columns.
The number of unique values for each column is calculated, and those columns that have a number of unique values less than 1 percent of the rows are identified. In this case, 11 columns.
The identified columns are then removed from the DataFrame, and the number of rows and columns in the DataFrame are reported to confirm the change.
(937, 50) [21, 22, 24, 25, 26, 32, 36, 38, 39, 45, 49] (937, 39)
Identify Rows That Contain Duplicate Data
Rows that have identical data are probably useless, if not dangerously misleading during model evaluation.
Here, a duplicate row is a row where each value in each column for that row appears in identically the same order (same column values) in another row.
From a probabilistic perspective, you can think of duplicate data as adjusting the priors for a class label or data distribution. This may help an algorithm like Naive Bayes if you wish to purposefully bias the priors. Typically, this is not the case and machine learning algorithms will perform better by identifying and removing rows with duplicate data.
From an algorithm evaluation perspective, duplicate rows will result in misleading performance. For example, if you are using a train/test split or k-fold cross-validation, then it is possible for a duplicate row or rows to appear in both train and test datasets and any evaluation of the model on these rows will be (or should be) correct. This will result in an optimistically biased estimate of performance on unseen data.
If you think this is not the case for your dataset or chosen model, design a controlled experiment to test it. This could be achieved by evaluating model skill with the raw dataset and the dataset with duplicates removed and comparing performance. Another experiment might involve augmenting the dataset with different numbers of randomly selected duplicate examples.
The pandas function duplicated() will report whether a given row is duplicated or not. All rows are marked as either False to indicate that it is not a duplicate or True to indicate that it is a duplicate. If there are duplicates, the first occurrence of the row is marked False (by default), as we might expect.
The example below checks for duplicates.
# locate rows of duplicate data from pandas import read_csv # define the location of the dataset path = 'https://raw.githubusercontent.com/jbrownlee/Datasets/master/iris.csv' # load the dataset df = read_csv(path, header=None) # calculate duplicates dups = df.duplicated() # report if there are any duplicates print(dups.any()) # list all duplicate rows print(df[dups])
Running the example first loads the dataset, then calculates row duplicates.
First, the presence of any duplicate rows is reported, and in this case, we can see that there are duplicates (True).
Then all duplicate rows are reported. In this case, we can see that three duplicate rows that were identified are printed.
True 0 1 2 3 4 34 4.9 3.1 1.5 0.1 Iris-setosa 37 4.9 3.1 1.5 0.1 Iris-setosa 142 5.8 2.7 5.1 1.9 Iris-virginica
Delete Rows That Contain Duplicate Data
Rows of duplicate data should probably be deleted from your dataset prior to modeling.
There are many ways to achieve this, although Pandas provides the drop_duplicates() function that achieves exactly this.
The example below demonstrates deleting duplicate rows from a dataset.
# delete rows of duplicate data from the dataset from pandas import read_csv # define the location of the dataset path = 'https://raw.githubusercontent.com/jbrownlee/Datasets/master/iris.csv' # load the dataset df = read_csv(path, header=None) print(df.shape) # delete duplicate rows df.drop_duplicates(inplace=True) print(df.shape)
Running the example first loads the dataset and reports the number of rows and columns.
Next, the rows of duplicated data are identified and removed from the DataFrame. Then the shape of the DataFrame is reported to confirm the change.
(150, 5) (147, 5)
Further Reading
This section provides more resources on the topic if you are looking to go deeper.
Tutorials
APIs
- numpy.unique API.
- pandas.DataFrame.nunique API.
- pandas.DataFrame.drop API.
- pandas.DataFrame.duplicated API.
- pandas.DataFrame.drop_duplicates API.
Summary
In this tutorial, you discovered basic data cleaning you should always perform on your dataset.
Specifically, you learned:
- How to identify and remove column variables that only have a single value.
- How to identify and consider column variables with very few unique values.
- How to identify and remove rows that contain duplicate observations.
Do you have any questions?
Ask your questions in the comments below and I will do my best to answer.
The post Basic Data Cleaning for Machine Learning (That You Must Perform) appeared first on Machine Learning Mastery.