Author: Jason Brownlee
Machine learning and deep learning methods are often reported to be the key solution to all predictive modeling problems.
An important recent study evaluated and compared the performance of many classical and modern machine learning and deep learning methods on a large and diverse set of more than 1,000 univariate time series forecasting problems.
The results of this study suggest that simple classical methods, such as linear methods and exponential smoothing, outperform complex and sophisticated methods, such as decision trees, Multilayer Perceptrons (MLP), and Long Short-Term Memory (LSTM) network models.
These findings highlight the requirement to both evaluate classical methods and use their results as a baseline when evaluating any machine learning and deep learning methods for time series forecasting in order demonstrate that their added complexity is adding skill to the forecast.
In this post, you will discover the important findings of this recent study evaluating and comparing the performance of a classical and modern machine learning methods on a large and diverse set of time series forecasting datasets.
After reading this post, you will know:
- Classical methods like ETS and ARIMA out-perform machine learning and deep learning methods for one-step forecasting on univariate datasets.
- Classical methods like Theta and ARIMA out-perform machine learning and deep learning methods for multi-step forecasting on univariate datasets.
- Machine learning and deep learning methods do not yet deliver on their promise for univariate time series forecasting, and there is much work to do.
Let’s get started.
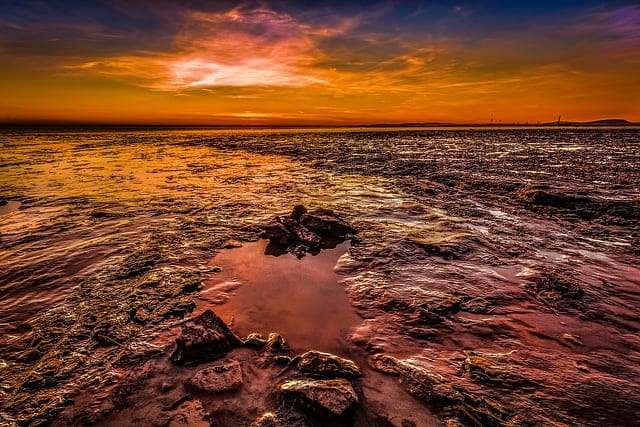
Findings Comparing Classical and Machine Learning Methods for Time Series Forecasting
Photo by Lyndon Hatherall, some rights reserved.
Overview
Spyros Makridakis, et al. published a study in 2018 titled “Statistical and Machine Learning forecasting methods: Concerns and ways forward.”
In this post, we will take a close look at the study by Makridakis, et al. that carefully evaluated and compared classical time series forecasting methods to the performance of modern machine learning methods.
This post is divided into seven sections; they are:
- Study Motivation
- Time Series Datasets
- Time Series Forecasting Methods
- Data Preparation
- One-Step Forecasting Results
- Multi-Step Forecasting Results
- Outcomes
Study Motivation
The goal of the study was to clearly demonstrate the capability of a suite of different machine learning methods as compared to classical time series forecasting methods on a very large and diverse collection of univariate time series forecasting problems.
The study was a response to the increasing number of papers and claims that machine learning and deep learning methods offer superior results for time series forecasting with little objective evidence.
Literally hundreds of papers propose new ML algorithms, suggesting methodological advances and accuracy improvements. Yet, limited objective evidence is available regarding their relative performance as a standard forecasting tool.
— Statistical and Machine Learning forecasting methods: Concerns and ways forward, 2018.
The authors clearly lay out three issues with the flood of claims; they are:
- Their conclusions are based on a few, or even a single time series, raising questions about the statistical significance of the results and their generalization.
- The methods are evaluated for short-term forecasting horizons, often one-step-ahead, not considering medium and long-term ones.
- No benchmarks are used to compare the accuracy of ML methods versus alternative ones.
As a response, the study includes eight classical methods and 10 machine learning methods evaluated using one-step and multiple-step forecasts across a collection of 1,045 monthly time series.
Although not definitive, the results are intended to be objective and robust.
Need help with Deep Learning for Time Series?
Take my free 7-day email crash course now (with sample code).
Click to sign-up and also get a free PDF Ebook version of the course.
Time Series Datasets
The time series datasets used in the study were drawn from the time series datasets used in the M3-Competition.
The M3-Competition was the third in a series of competitions that sought to discover exactly what algorithms perform well in practice on real time series forecasting problems. The results of the competition were published in the 2000 paper titled “The M3-Competition: Results, Conclusions and Implications.”
The datasets used in the competition were drawn from a wide range of industries and had a range of different time intervals, from hourly to annual.
The 3003 series of the M3-Competition were selected on a quota basis to include various types of time series data (micro, industry, macro, etc.) and different time intervals between successive observations (yearly, quarterly, etc.).
The table below, taken from the paper, provides a summary of the 3,003 datasets used in the competition.

Table of Datasets, Industry and Time Interval Used in the M3-Competition
Taken from “The M3-Competition: Results, Conclusions and Implications.”
The finding of the competition was that simpler time series forecasting methods outperform more sophisticated methods, including neural network models.
This study, the previous two M-Competitions and many other empirical studies have proven, beyond the slightest doubt, that elaborate theoretical constructs or more sophisticated methods do not necessarily improve post-sample forecasting accuracy, over simple methods, although they can better fit a statistical model to the available historical data.
— The M3-Competition: Results, Conclusions and Implications, 2000.
The more recent study that we are reviewing in this post that evaluate machine learning methods selected a subset of 1,045 time series with a monthly interval from those used in the M3 competition.
… evaluate such performance across multiple forecasting horizons using a large subset of 1045 monthly time series used in the M3 Competition.
— Statistical and Machine Learning forecasting methods: Concerns and ways forward, 2018.
Time Series Forecasting Methods
The study evaluates the performance of eight classical (or simpler) methods and 10 machine learning methods.
… of eight traditional statistical methods and eight popular ML ones, […], plus two more that have become popular during recent years.
— Statistical and Machine Learning forecasting methods: Concerns and ways forward, 2018.
The eight classical methods evaluated were as follows:
- Naive 2, which is actually a random walk model adjusted for season.
- Simple Exponential Smoothing.
- Holt.
- Damped exponential smoothing.
- Average of SES, Holt, and Damped.
- Theta method.
- ARIMA, automatic.
- ETS, automatic.
A total of eight machine learning methods were used in an effort to reproduce and compare to results presented in the 2010 paper “An Empirical Comparison of Machine Learning Models for Time Series Forecasting.”
They were:
- Multi-Layer Perceptron (MLP)
- Bayesian Neural Network (BNN)
- Radial Basis Functions (RBF)
- Generalized Regression Neural Networks (GRNN), also called kernel regression
- K-Nearest Neighbor regression (KNN)
- CART regression trees (CART)
- Support Vector Regression (SVR)
- Gaussian Processes (GP)
An additional two ‘modern‘ neural network algorithms were also added to the list given the recent rise in their adoption; they were:
- Recurrent Neural Network (RNN)
- Long Short-Term Memory (LSTM)
Data Preparation
A careful data preparation methodology was used, again, based on the methodology described in the 2010 paper “An Empirical Comparison of Machine Learning Models for Time Series Forecasting.”
In that paper, each time series was adjusted using a power transform, deseasonalized and detrended.
[…] before computing the 18 forecasts, they preprocessed the series in order to achieve stationarity in their mean and variance. This was done using the log transformation, then deseasonalization and finally scaling, while first differences were also considered for removing the component of trend.
— Statistical and Machine Learning forecasting methods: Concerns and ways forward, 2018.
Inspired by these operations, variations of five different data transforms were applied for an MLP for one-step forecasting and their results were compared. The five transforms were:
- Original data.
- Box-Cox Power Transform.
- Deseasonalizing the data.
- Detrending the data.
- All three transforms (power, deseasonalize, detrend).
Generally, it was found that the best approach was to apply a power transform and deseasonalize the data, and perhaps detrend the series as well.
The best combination according to sMAPE is number 7 (Box-Cox transformation, deseasonalization) while the best one according to MASE is number 10 (Box-Cox transformation, deseasonalization and detrending)
— Statistical and Machine Learning forecasting methods: Concerns and ways forward, 2018.
One-Step Forecasting Results
All models were evaluated using one-step time series forecasting.
Specifically, the last 18 time steps were used as a test set, and models were fit on all remaining observations. A separate one-step forecast was made for each of the 18 observations in the test set, presumably using a walk-forward validation method where true observations were used as input in order to make each forecast.
The forecasting model was developed using the first n – 18 observations, where n is the length of the series. Then, 18 forecasts were produced and their accuracy was evaluated compared to the actual values not used in developing the forecasting model.
— Statistical and Machine Learning forecasting methods: Concerns and ways forward, 2018.
Reviewing the results, the MLP and BNN were found to achieve the best performance from all of the machine learning methods.
The results […] show that MLP and BNN outperform the remaining ML methods.
— Statistical and Machine Learning forecasting methods: Concerns and ways forward, 2018.
A surprising result was that RNNs and LSTMs were found to perform poorly.
It should be noted that RNN is among the less accurate ML methods, demonstrating that research progress does not necessarily guarantee improvements in forecasting performance. This conclusion also applies in the performance of LSTM, another popular and more advanced ML method, which does not enhance forecasting accuracy too.
— Statistical and Machine Learning forecasting methods: Concerns and ways forward, 2018.
Comparing the performance of all methods, it was found that the machine learning methods were all out-performed by simple classical methods, where ETS and ARIMA models performed the best overall.
This finding confirms the results from previous similar studies and competitions.
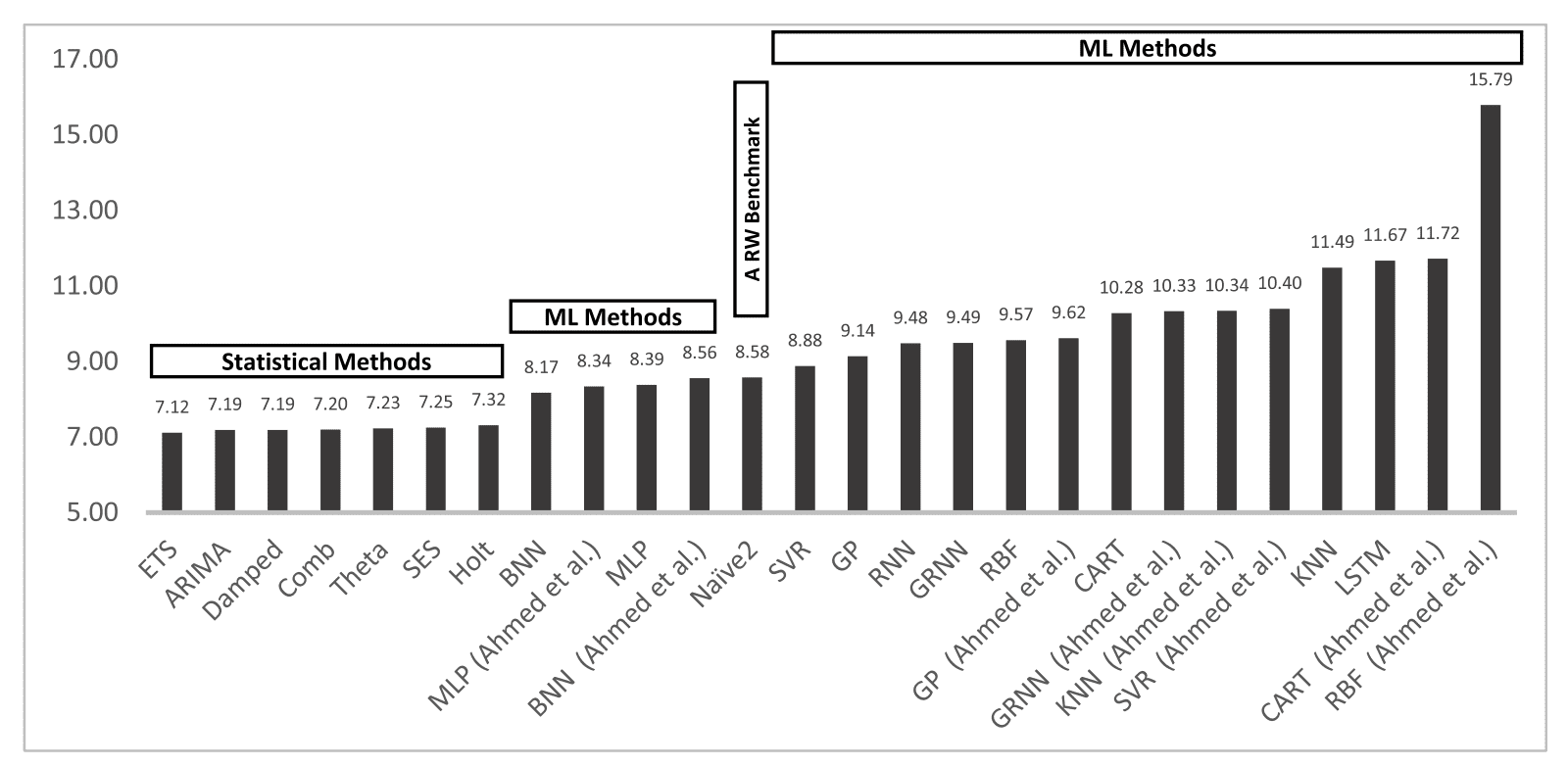
Bar Chart Comparing Model Performance (sMAPE) for One-Step Forecasts
Taken from “Statistical and Machine Learning forecasting methods: Concerns and ways forward.”
Multi-Step Forecasting Results
Multi-step forecasting involves predicting multiple steps ahead of the last known observation.
Three approaches to multi-step forecasting were evaluated for the machine learning methods; they were:
- Iterative forecasting
- Direct forecasting
- Multi-neural network forecasting
The classical methods were found to outperform the machine learning methods again.
In this case, methods such as Theta, ARIMA, and a combination of exponential smoothing (Comb) were found to achieve the best performance.
In brief, statistical models seem to generally outperform ML methods across all forecasting horizons, with Theta, Comb and ARIMA being the dominant ones among the competitors according to both error metrics examined.
— Statistical and Machine Learning forecasting methods: Concerns and ways forward, 2018.
Outcomes
The study provides important supporting evidence that classical methods may dominate univariate time series forecasting, at least on the types of forecasting problems evaluated.
The study demonstrates the worse performance and the increase in computational cost of machine learning and deep learning methods for univariate time series forecasting for both one-step and multi-step forecasts.
These findings strongly encourage the use of classical methods, such as ETS, ARIMA, and others as a first step before more elaborate methods are explored, and requires that the results from these simpler methods be used as a baseline in performance that more elaborate methods must clear in order to justify their usage.
It also highlights the need to not just consider the careful use of data preparation methods, but to actively test multiple different combinations of data preparation schemes for a given problem in order to discover what works best, even in the case of classical methods.
Machine learning and deep learning methods may still achieve better performance on specific univariate time series problems and should be evaluated.
The study does not look at more complex time series problems, such as those datasets with:
- Complex irregular temporal structures.
- Missing observations
- Heavy noise.
- Complex interrelationships between multiple variates.
The study concludes with an honest puzzlement at why machine learning methods perform so poorly in practice, given their impressive performance in other areas of artificial intelligence.
The most interesting question and greatest challenge is to find the reasons for their poor performance with the objective of improving their accuracy and exploiting their huge potential. AI learning algorithms have revolutionized a wide range of applications in diverse fields and there is no reason that the same cannot be achieved with the ML methods in forecasting. Thus, we must find how to be applied to improve their ability to forecast more accurately.
— Statistical and Machine Learning forecasting methods: Concerns and ways forward, 2018.
Comments are made by the authors regarding LSTMs and RNNs, that are generally believed to be the deep learning approach for sequence prediction problems in general, and in this case their clearly poor performance in practice.
[…] one would expect RNN and LSTM, which are more advanced types of NNs, to be far more accurate than the ARIMA and the rest of the statistical methods utilized.
— Statistical and Machine Learning forecasting methods: Concerns and ways forward, 2018.
They comment that LSTMs appear to be more suited at fitting or overfitting the training dataset rather than forecasting it.
Another interesting example could be the case of LSTM that compared to simpler NNs like RNN and MLP, report better model fitting but worse forecasting accuracy
— Statistical and Machine Learning forecasting methods: Concerns and ways forward, 2018.
There is work to do and machine learning methods and deep learning methods hold the promise of better learning time series data than classical statistical methods, and even doing so directly on the raw observations via automatic feature learning.
Given their ability to learn, ML methods should do better than simple benchmarks, like exponential smoothing. Accepting the problem is the first step in devising workable solutions and we hope that those in the field of AI and ML will accept the empirical findings and work to improve the forecasting accuracy of their methods.
— Statistical and Machine Learning forecasting methods: Concerns and ways forward, 2018.
Further Reading
This section provides more resources on the topic if you are looking to go deeper.
- Makridakis Competitions, Wikipedia
- The M3-Competition: Results, Conclusions and Implications, 2000.
- The M4 Competition: Results, findings, conclusion and way forward, 2018.
- Statistical and Machine Learning forecasting methods: Concerns and ways forward, 2018.
- An Empirical Comparison of Machine Learning Models for Time Series Forecasting, 2010.
Summary
In this post, you discovered the important findings of a recent study evaluating and comparing the performance of classical and modern machine learning methods on a large and diverse set of time series forecasting datasets.
Specifically, you learned:
- Classical methods like ETS and ARIMA out-perform machine learning and deep learning methods for one-step forecasting on univariate datasets.
- Classical methods like Theta and ARIMA out-perform machine learning and deep learning methods for multi-step forecasting on univariate datasets.
- Machine learning and deep learning methods do not yet deliver on their promise for univariate time series forecasting and there is much work to do.
Do you have any questions?
Ask your questions in the comments below and I will do my best to answer.
The post Results From Comparing Classical and Machine Learning Methods for Time Series Forecasting appeared first on Machine Learning Mastery.