Author:
Seeing music. Predicting earthquake aftershocks. Finding emojis in real life. These are just a few examples of how researchers, engineers and user-experience (UX) professionals made imaginative ideas real. They made it happen using tools and techniques developed by Google’s People + AI Research (PAIR) team in 2018.
We founded PAIR in 2017 to conduct research, create design frameworks and build new technologies that help make partnerships between humans and artificial intelligence productive, enjoyable and fair. One of our main goals is to create easy-to-use tools to visualize machine learning (ML) datasets and train ML models (the mathematical equations that represent the steps a machine will complete to make a decision) in browsers. Put simply, this means anyone with an internet connection can now use ML.
Here’s what PAIR has accomplished over the past year—and here’s how engineers and UX teams can put our resources to use in 2019 and beyond.
Creating a design library—and learning how to design for AI
In January, we launched a library of user-experience articles and case studies on Google Design. These show how Google makes decisions to balance our users’ needs for familiarity and trust with new functionality and experiences enabled by AI. The case studies go behind the scenes to show how Google teams developed user experiences for applications, like the fun mobile game Emoji Scavenger Hunt.
In these articles, practicing user-experience designers offer clear how-tos. They address challenges in designing for AI, such as balancing how to design for habits like swiping or scrolling in certain directions, and building personalized experiences for individual users. We know we don’t have all the answers, so we also seek advice from outside experts, like Paola Antonelli, Senior Curator of Architecture and Design at New York’s Museum of Modern Art (MoMA), who answered our team’s questions on how to use AI as a design material itself.
Talking about AI across disciplines
A key part of our process is partnering with domain experts in other fields. For example, this year we worked with Harvard’s Brendan Meade and the University of Connecticut’s Phoebe de Vries on a model for predicting and visualizing earthquake aftershocks. This project led to a state-of-the-art model for aftershock prediction–and, intriguingly, our analysis of the AI suggested new, unexpected directions for human researchers to investigate.
In March, we hosted our first UX symposium in Zurich, featuring external researchers and industry professionals. And in May, we held a panel at I/O, “AI for Everyone,” featuring Google engineering leaders with a spectrum of expertise, from cloud computing to climate science, to discuss fair and inclusive AI in these fields.
We’re also dedicated to translating the complicated language behind AI for everyone who uses it, even if they’re not engineers. Since June, our first PAIR writer-in-residence, tech journalist David Weinberger, has been embedded in PAIR’s Cambridge, Mass. lab. He’s explaining key AI concepts, like classification and confidence levels, and timely topics like fairness in machine learning, for non-technical audiences.
New open-source tools for engineers, UXers and beyond
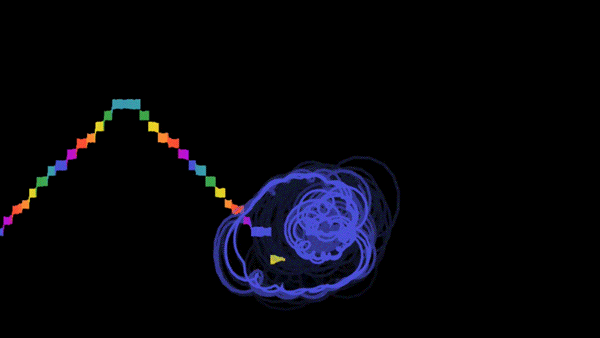
Using TensorFlow.js, an open-source Javascript library created by PAIR, and other software, a group of musicians, designers, engineers and the Google Creative Lab created Seeing Music, which makes it possible to visualize subtle textures in sound.
We believe in applying deep insights to invent, and open-source, new technologies that can be used by engineers, UX professionals, and other stakeholders who may not be experts in ML.
So we started TensorFlow.js, a pure Javascript library that extends TensorFlow into the browser. Since open-sourcing TensorFlow.js in March, we’ve seen a variety of applications–including a set of accessible creative tools for drawing, making music and more, designed by Google’s Creative Lab with collaborators from the accessibility community.
Our PAIR team also built the What-If Tool, released this fall, so professionals building ML systems don’t have to write a single line of code to answer “what if” questions such as: “What if I changed data points, how would this affect my model’s predictions? Does it perform differently for various groups–for example, historically marginalized people?” Our tool makes it possible to simply click a button to visualize and inspect alternative scenarios.
Also this year, our team developed and open-sourced a new technique for helping people more easily understand the inner workings of neural networks in terms of simple, human-understandable concepts – like showing how AI can recognize images of zebras by their stripes.
In 2019, we’re excited to expand PAIR’s work further with global audiences of engineers and user-experience designers–and everyday users. For more resources, updates and information on our research, head to PAIR’s website.