Author: Jason Brownlee
Computer vision is perhaps one area that has been most impacted by developments in deep learning.
It can be difficult to both develop and to demonstrate competence with deep learning for problems in the field of computer vision. It is not clear how to get started, what the most important techniques are, and the types of problems and projects that can best highlight the value that deep learning can bring to the field.
On approach is to systematically develop, and at the same time demonstrate competence with, data handling, modeling techniques, and application domains and present your results in a public portfolio of completed projects. This approach allows you to compound your skills from project to project. It also provides the basis for real projects that can be presented and discussed with prospective employers in order to demonstrate your capabilities.
In this post, you will discover how to develop and demonstrate competence in deep learning applied to problems in computer vision.
After reading this post, you will know:
- Developing a portfolio of completed small projects can both be leveraged on new projects in the future and demonstrate your competence with deep learning for computer vision projects.
- Projects can be kept small in scope, although they can still demonstrate a systematic approach to problem-solving and the development of skillful models.
- A three-level competence framework can be followed that includes data handling competence, technique competence, and application competence.
Let’s get started.
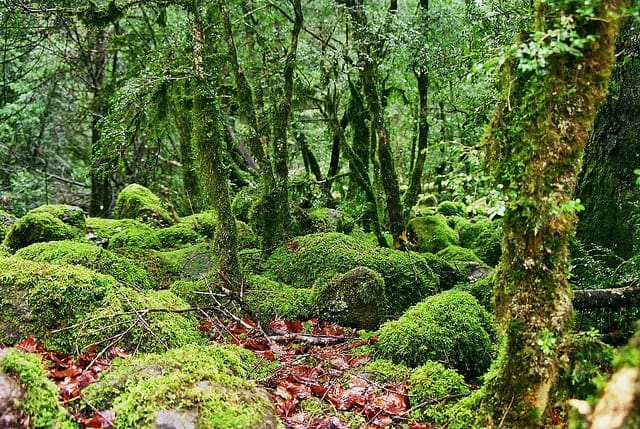
How to Develop and Demonstrate Competence With Deep Learning for Computer Vision
Photo by Sole Perez, some rights reserved.
Overview
This tutorial is divided into three parts; they are:
- Deep Learning for Computer Vision
- Develop a Portfolio of Small Projects
- Deep Learning for Computer Vision Competence Framework
Deep Learning for Computer Vision
Perhaps one domain that has been the most impacted by developments in deep learning is computer vision.
Computer vision is a subfield of artificial intelligence concerned with understanding data in images, such as photos and videos.
Computer vision tasks such as recognizing handwritten digits and objects in photographs were some of the early case studies demonstrating the capability of modern deep learning techniques achieving state-of-the-art results.
As a practitioner, you may wish to develop and demonstrate your skills with deep learning in computer vision.
This does assume a few things, such as:
- You are familiar with applied machine learning, meaning that you are able to work through a predictive modeling project end-to-end and deliver a skillful model.
- You are familiar with deep learning techniques, meaning that you know the difference between the main methods and when to use them.
This does not mean that you are an expert, only that you have a working knowledge and are able to wok through problems systematically.
As a machine learning or even deep learning practitioner, how can you show competence with computer vision applications?
Develop a Portfolio of Small Projects
Competence with deep learning for computer vision can be developed and demonstrated using a project-based approach.
Specifically, the skills can be built and demonstrated incrementally by completing and presenting small projects that use deep learning techniques on computer vision problems.
This requires you to develop a portfolio of completed projects. A portfolio helps you in two specific ways:
- Skill Development: The code and findings from the projects in the portfolio can be leveraged by you on future projects, accelerating your progress and allowing you to take on larger and more challenging projects.
- Skill Demonstration: The public presentation of the projects provides a demonstration of your capabilities, providing the basis for discussion of APIs, model selection, and design decisions with prospective employers.
Projects can be focused on standard and publicly available computer vision datasets, such as those developed and hosted by academics or those used in machine learning competitions.
Projects can be completed in a systematic manner, including aspects such as clear problem definition, review of relevant literature and models, model development and tuning, and the presentation of results and findings in a report, notebook, or even slideshow presentation format.
Projects are small, meaning that they can be completed in a workday, perhaps spread over a number of nights and weekends. This is important as it limits the scope of the project to focus on workflow and delivering a skillful result, rather than developing a state-of-the-art result.
Deep Learning for Computer Vision Competence Framework
Projects can be selected carefully in such a way to both build in terms of challenge or complexity and in terms of leverage or skill development.
Below is a three-level framework for developing and demonstrating competence with deep learning for computer vision, intended for practitioners already familiar with the basics of applied machine learning and the basics of deep learning:
- Level 1: Data Handling Competence. That you know how to load and manipulate image data.
- Level 2: Technique Competence. That you know how to define, fit, and tune convolutional neural networks.
- Level 3: Application Competence. That you can develop skillful deep learning models for common computer vision problems.
Level 1: Data Handling Competence
Data handling competence refers to the ability to load and transform data.
This includes basic data I/O operations such as loading and saving image or video data.
Most importantly, it involves using standard APIs to manipulate image data in ways that may be useful when preparing data for molding with deep learning neural networks.
Examples include:
- Image resizing and interpolation.
- Image blurring and sharpening.
- Image affine transforms.
- Image whitening and thresholding.
Data handling could be demonstrated with one of many image handling APIs, such as:
It may include the basic data handing capability of machine learning and deep learning libraries, such as:
What are your favorite image handling APIs in Python?
Let me know in the comments below.
Level 2: Technique Competence
Technique competence refers to the ability to use the specific deep learning models and methods that are used for computer vision problems.
This includes from a high-level the three main classes of methods:
- Multilayer Perceptrons, or MLPs.
- Convolutional Neural Networks, or CNNs.
- Recurrent Neural Networks, such as the Long Short-Term Memory Network, or LSTM.
More specifically, this requires a demonstration of strong skills with how to configure and get the most of the layers used in a CNN, such as:
- Convolutional Layers.
- Pooling Layers.
- Patterns of using Layers.
This may also include skill with some general classes of effective models, such as:
- ImageNet CNNs such as AlexNet, VGG, ResNet, Inception, etc.
- CNN-LSTMs, LSTM-CNNs, etc.
- R-CNNs, YOLO, etc.
What are your favorite deep learning techniques for computer vision?
Let me know in the comments below.
Level 3: Application Competence
Application competence refers to the ability to work through a specific computer vision problem and use deep learning methods to deliver a skillful model.
A skillful model means a model that is capable of making predictions that have better performance than a naive baseline method. It does not mean achieving state-of-the-art results and replicating a model and results in a paper, although they are fine project ideas if they are within scope of a small project.
The project should be completed systematically, including most if not all of the following steps:
- Problem Description. Describe the predictive modeling problem, including the domain and relevant background.
- Literature Review. Describe standard or common approaches to solving the problem using deep learning methods as described in seminal and/or recent research papers.
- Summarize Data. Describe the available data, including statistical summaries and data visualization.
- Evaluate Models. Spot-check a suite of model types, configurations, data preparation schemes, and more in order to narrow down what works well on the problem.
- Improve Performance. Improve the performance of the model or models that work well with hyperparameter tuning and perhaps ensemble methods.
- Present Results. Present the findings of the project.
A step before this process, step zero, might be to choose a publicly available dataset appropriate for the project.
The backbone of deep learning for computer vision is image classification, commonly referred to as image recognition or object detection. This involves predicting a class label given an image, often a photograph.
Problems of this type should be the focus.
Two standard computer vision datasets of this type include:
- Classifying handwritten digits (e.g. MNIST and SVHN).
- Classifying photos of objects (e.g. CIFAR-10 and CIFAR-100).
- Classifying photos of faces (e.g. VGGFace2)
A related computer vision task is identifying the location of one or more objects within photographs, also referred to as object recognition or object localization or segmentation.
- Object Recognition and Localization (e.g. COCO)
There are also tasks that involve a mixture of computer vision and natural language processing, for example:
- Photo Captioning (e.g. Flickr8k)
Finally, there are computer vision tasks that can be performed using manipulations of existing standard datasets or catalogs of photos, such as:
- Photo Colorization.
- Photo Reconstruction.
- Photo Super-Resolution.
- Photo Synthesis (e.g. deep fakes).
What are your favorite applications of deep learning for computer vision?
Let me know in the comments below.
Further Reading
This section provides more resources on the topic if you are looking to go deeper.
APIs
- Multi-dimensional image processing (scipy.ndimage) API
- Multidimensional image processing (scipy.ndimage) Tutorial
- scikit-image
- Pillow
- OpenCV
- OpenCV Python Tutorials
- sklearn.feature_extraction: Feature Extraction API
- Keras Image Preprocessing API
Datasets
Articles
- Computer Vision, Wikipedia.
- Film colorization, Wikipedia.
- Super-resolution imaging, Wikipedia.
- Digital photograph restoration, Wikipedia.
- Deefake, Wikipedia.
Summary
In this post, you discovered how to develop and demonstrate competence in deep learning applied to problems in computer vision.
Specifically, you learned:
- Developing a portfolio of completed small projects can both be leveraged on new projects in the future and demonstrate your competence with deep learning for computer vision projects.
- Projects can be kept small in scope although they can still demonstrate a systematic approach to problem-solving and the development of skillful models.
- A three-level competence framework can be followed that includes data handling competence, technique competence, and application competence.
Do you have any questions?
Ask your questions in the comments below and I will do my best to answer.
The post How to Develop and Demonstrate Competence With Deep Learning for Computer Vision appeared first on Machine Learning Mastery.