Author: Jason Brownlee
The field of computer vision is shifting from statistical methods to deep learning neural network methods.
There are still many challenging problems to solve in computer vision. Nevertheless, deep learning methods are achieving state-of-the-art results on some specific problems.
It is not just the performance of deep learning models on benchmark problems that is most interesting; it is the fact that a single model can learn meaning from images and perform vision tasks, obviating the need for a pipeline of specialized and hand-crafted methods.
In this post, you will discover nine interesting computer vision tasks where deep learning methods are achieving some headway.
Let’s get started.
Overview
In this post, we will look at the following computer vision problems where deep learning has been used:
- Image Classification
- Image Classification With Localization
- Object Detection
- Object Segmentation
- Image Style Transfer
- Image Colorization
- Image Reconstruction
- Image Super-Resolution
- Image Synthesis
- Other Problems
Note, when it comes to the image classification (recognition) tasks, the naming convention from the ILSVRC has been adopted. Although the tasks focus on images, they can be generalized to the frames of video.
I have tried to focus on the types of end-user problems that you may be interested in, as opposed to more academic sub-problems where deep learning does well.
Each example provides a description of the problem, an example, and references to papers that demonstrate the methods and results.
Do you have a favorite computer vision application for deep learning that is not listed?
Let me know in the comments below.
Image Classification
Image classification involves assigning a label to an entire image or photograph.
This problem is also referred to as “object classification” and perhaps more generally as “image recognition,” although this latter task may apply to a much broader set of tasks related to classifying the content of images.
Some examples of image classification include:
- Labeling an x-ray as cancer or not (binary classification).
- Classifying a handwritten digit (multiclass classification).
- Assigning a name to a photograph of a face (multiclass classification).
A popular example of image classification used as a benchmark problem is the MNIST dataset.
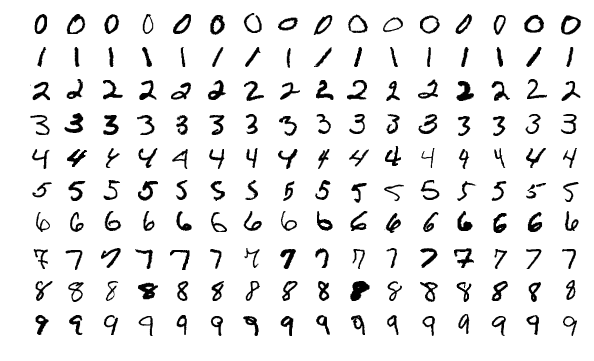
Example of Handwritten Digits From the MNIST Dataset
A popular real-world version of classifying photos of digits is The Street View House Numbers (SVHN) dataset.
For state-of-the-art results and relevant papers on these and other image classification tasks, see:
There are many image classification tasks that involve photographs of objects. Two popular examples include the CIFAR-10 and CIFAR-100 datasets that have photographs to be classified into 10 and 100 classes respectively.

Example of Photographs of Objects From the CIFAR-10 Dataset
The Large Scale Visual Recognition Challenge (ILSVRC) is an annual competition in which teams compete for the best performance on a range of computer vision tasks on data drawn from the ImageNet database. Many important advancements in image classification have come from papers published on or about tasks from this challenge, most notably early papers on the image classification task. For example:
- ImageNet Classification With Deep Convolutional Neural Networks, 2012.
- Very Deep Convolutional Networks for Large-Scale Image Recognition, 2014.
- Going Deeper with Convolutions, 2015.
- Deep Residual Learning for Image Recognition, 2015.
Image Classification With Localization
Image classification with localization involves assigning a class label to an image and showing the location of the object in the image by a bounding box (drawing a box around the object).
This is a more challenging version of image classification.
Some examples of image classification with localization include:
- Labeling an x-ray as cancer or not and drawing a box around the cancerous region.
- Classifying photographs of animals and drawing a box around the animal in each scene.
A classical dataset for image classification with localization is the PASCAL Visual Object Classes datasets, or PASCAL VOC for short (e.g. VOC 2012). These are datasets used in computer vision challenges over many years.
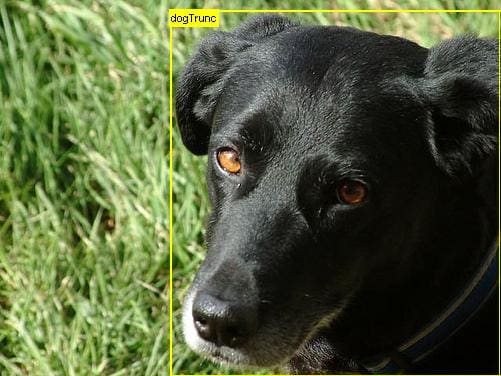
Example of Image Classification With Localization of a Dog from VOC 2012
The task may involve adding bounding boxes around multiple examples of the same object in the image. As such, this task may sometimes be referred to as “object detection.”
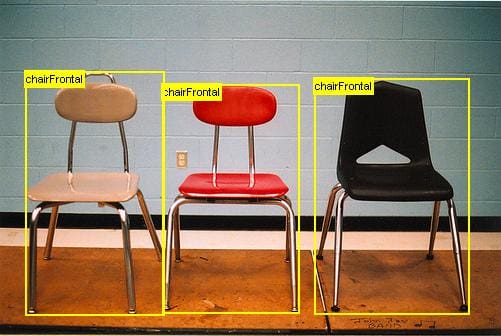
Example of Image Classification With Localization of Multiple Chairs From VOC 2012
The ILSVRC2016 Dataset for image classification with localization is a popular dataset comprised of 150,000 photographs with 1,000 categories of objects.
Some examples of papers on image classification with localization include:
- Selective Search for Object Recognition, 2013.
- Rich feature hierarchies for accurate object detection and semantic segmentation, 2014.
- Fast R-CNN, 2015.
Object Detection
Object detection is the task of image classification with localization, although an image may contain multiple objects that require localization and classification.
This is a more challenging task than simple image classification or image classification with localization, as often there are multiple objects in the image of different types.
Often, techniques developed for image classification with localization are used and demonstrated for object detection.
Some examples of object detection include:
- Drawing a bounding box and labeling each object in a street scene.
- Drawing a bounding box and labeling each object in an indoor photograph.
- Drawing a bounding box and labeling each object in a landscape.
The PASCAL Visual Object Classes datasets, or PASCAL VOC for short (e.g. VOC 2012), is a common dataset for object detection.
Another dataset for multiple computer vision tasks is Microsoft’s Common Objects in Context Dataset, often referred to as MS COCO.
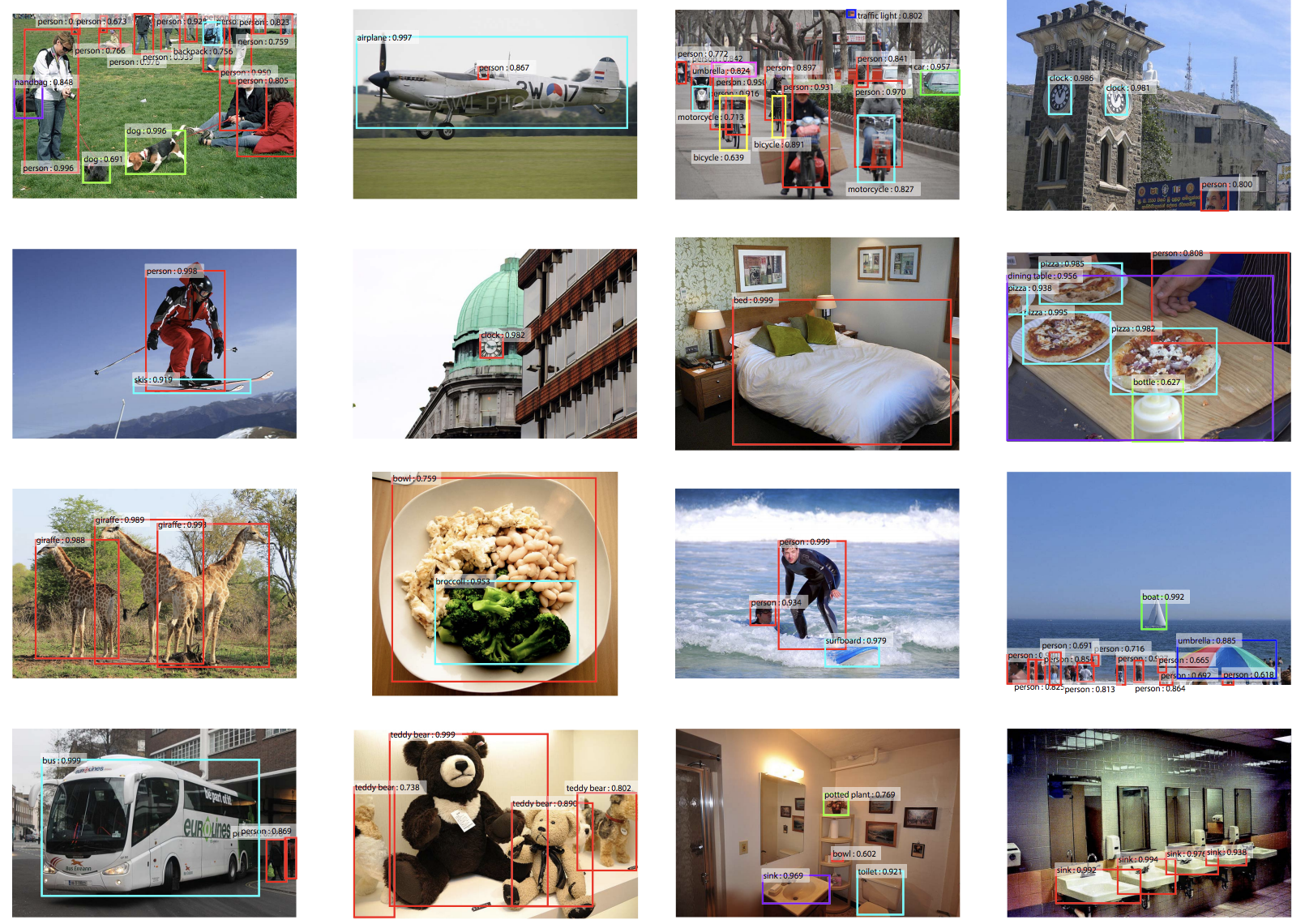
Example of Object Detection With Faster R-CNN on the MS COCO Dataset
Some examples of papers on object detection include:
- OverFeat: Integrated Recognition, Localization and Detection using Convolutional Networks, 2014.
- Faster R-CNN: Towards Real-Time Object Detection with Region Proposal Networks, 2015.
- You Only Look Once: Unified, Real-Time Object Detection, 2015.
Object Segmentation
Object segmentation, or semantic segmentation, is the task of object detection where a line is drawn around each object detected in the image. Image segmentation is a more general problem of spitting an image into segments.
Object detection is also sometimes referred to as object segmentation.
Unlike object detection that involves using a bounding box to identify objects, object segmentation identifies the specific pixels in the image that belong to the object. It is like a fine-grained localization.
More generally, “image segmentation” might refer to segmenting all pixels in an image into different categories of object.
Again, the VOC 2012 and MS COCO datasets can be used for object segmentation.
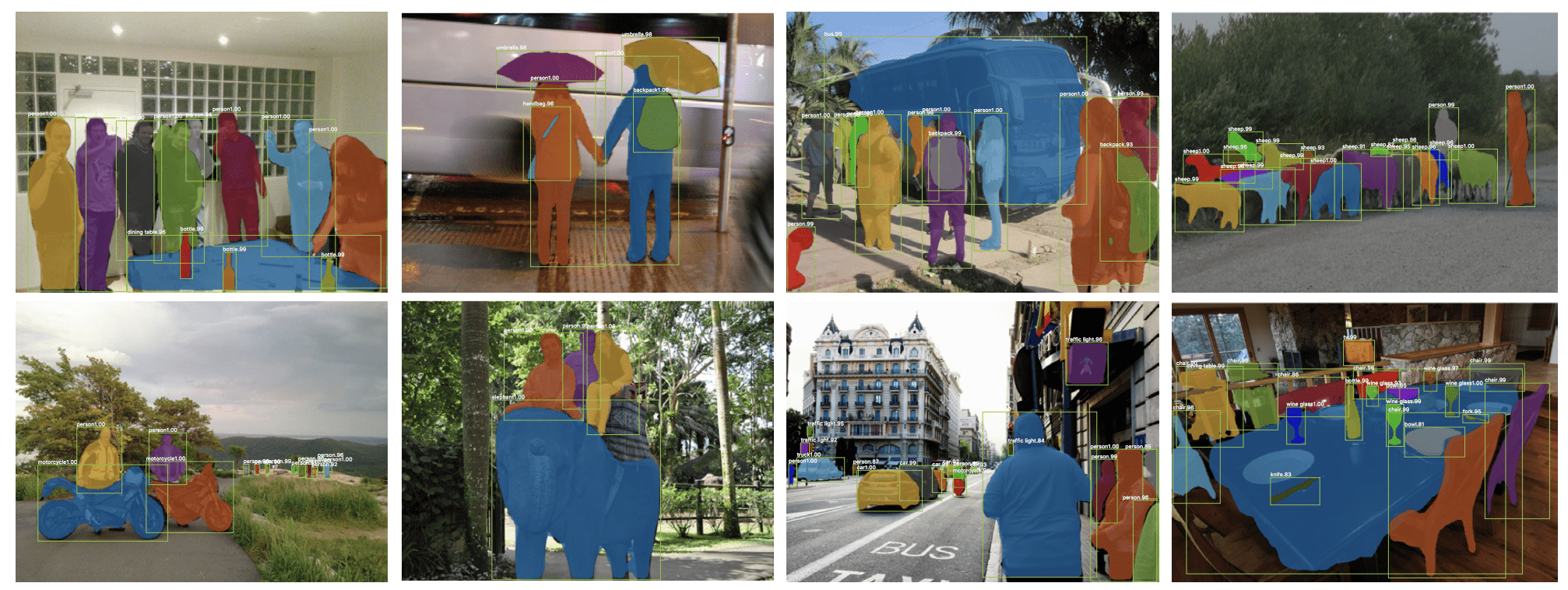
Example of Object Segmentation on the COCO Dataset
Taken from “Mask R-CNN”.
The KITTI Vision Benchmark Suite is another object segmentation dataset that is popular, providing images of streets intended for training models for autonomous vehicles.
Some example papers on object segmentation include:
- Simultaneous Detection and Segmentation, 2014.
- Fully Convolutional Networks for Semantic Segmentation, 2015.
- Hypercolumns for Object Segmentation and Fine-grained Localization, 2015.
- SegNet: A Deep Convolutional Encoder-Decoder Architecture for Image Segmentation, 2016.
- Mask R-CNN, 2017.
Style Transfer
Style transfer or neural style transfer is the task of learning style from one or more images and applying that style to a new image.
This task can be thought of as a type of photo filter or transform that may not have an objective evaluation.
Examples include applying the style of specific famous artworks (e.g. by Pablo Picasso or Vincent van Gogh) to new photographs.
Datasets often involve using famous artworks that are in the public domain and photographs from standard computer vision datasets.
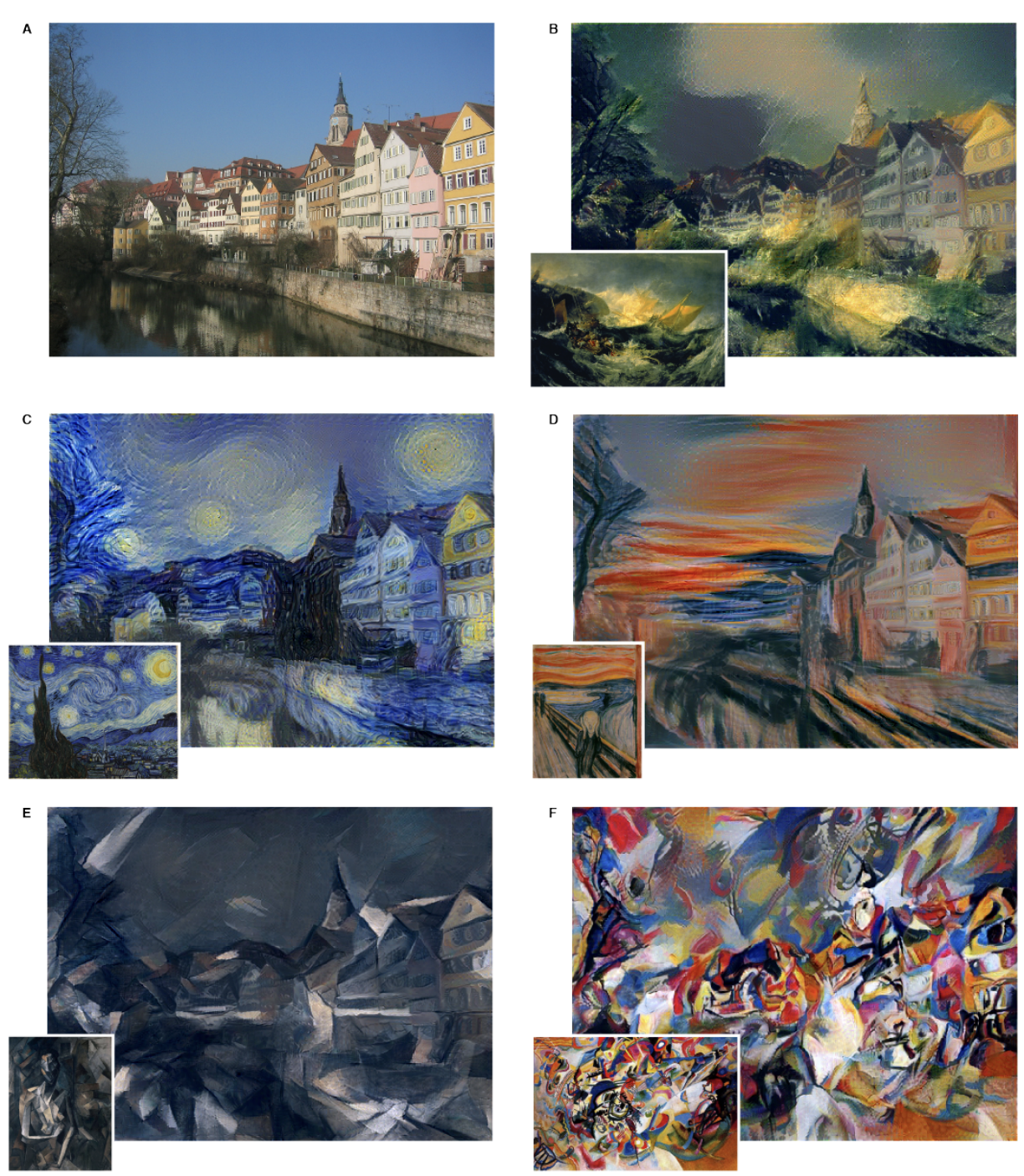
Example of Neural Style Transfer From Famous Artworks to a Photograph
Taken from “A Neural Algorithm of Artistic Style”
Some papers include:
- A Neural Algorithm of Artistic Style, 2015.
- Image Style Transfer Using Convolutional Neural Networks, 2016.
Image Colorization
Image colorization or neural colorization involves converting a grayscale image to a full color image.
This task can be thought of as a type of photo filter or transform that may not have an objective evaluation.
Examples include colorizing old black and white photographs and movies.
Datasets often involve using existing photo datasets and creating grayscale versions of photos that models must learn to colorize.
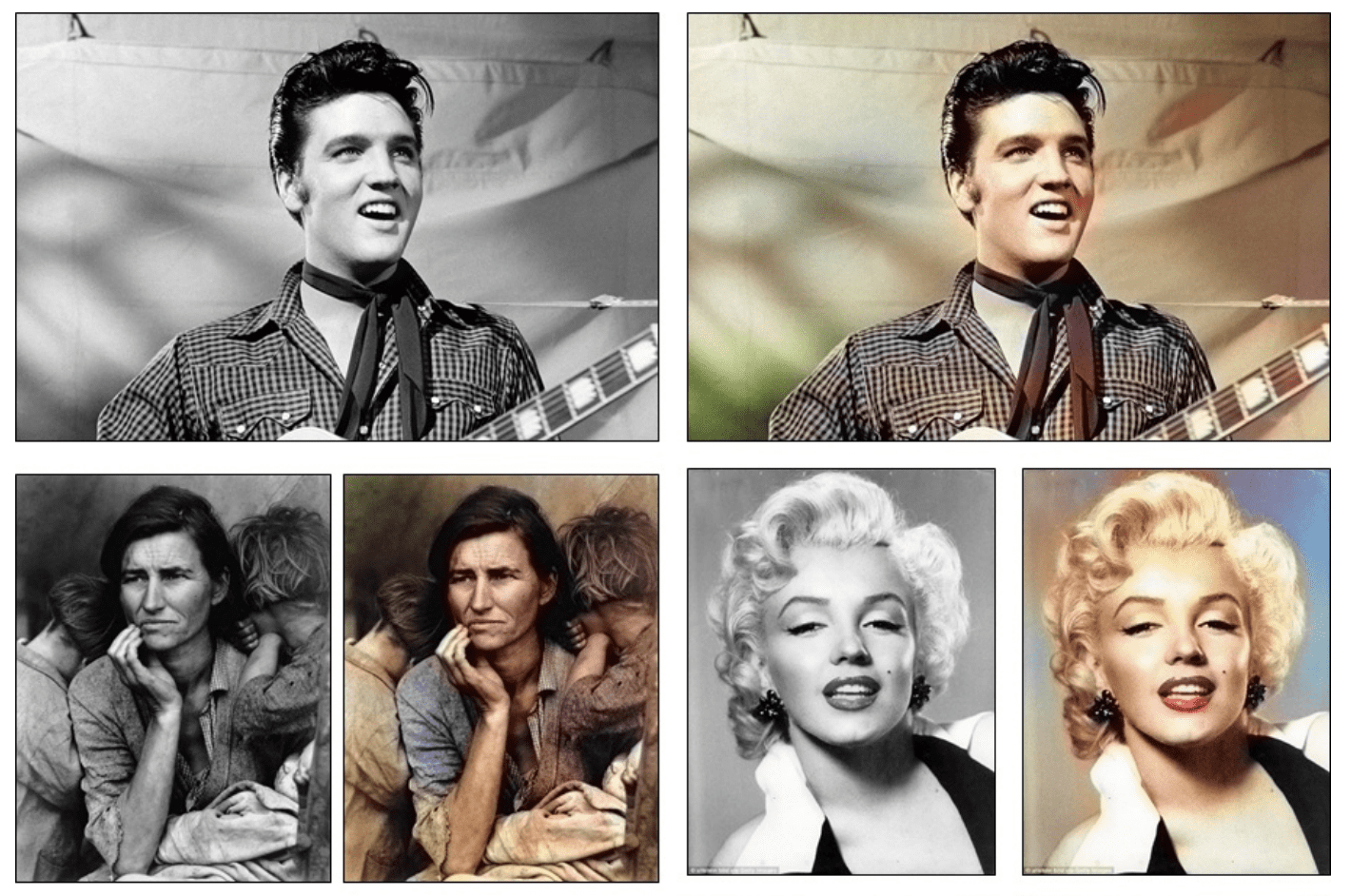
Examples of Photo Colorization
Taken from “Colorful Image Colorization”
Some papers include:
- Colorful Image Colorization, 2016.
- Let there be Color!: Joint End-to-end Learning of Global and Local Image Priors for Automatic Image Colorization with Simultaneous Classification, 2016.
- Deep Colorization, 2016.
Image Reconstruction
Image reconstruction and image inpainting is the task of filling in missing or corrupt parts of an image.
This task can be thought of as a type of photo filter or transform that may not have an objective evaluation.
Examples include reconstructing old, damaged black and white photographs and movies (e.g. photo restoration).
Datasets often involve using existing photo datasets and creating corrupted versions of photos that models must learn to repair.
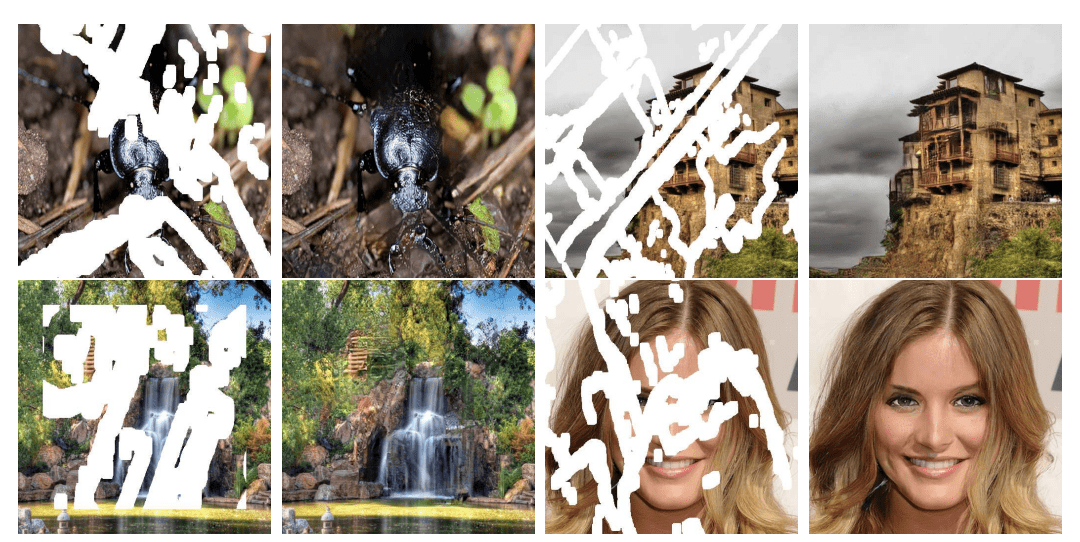
Example of Photo Inpainting.
Taken from “Image Inpainting for Irregular Holes Using Partial Convolutions”
Some papers include:
- Pixel Recurrent Neural Networks, 2016.
- Image Inpainting for Irregular Holes Using Partial Convolutions, 2018.
- Highly Scalable Image Reconstruction using Deep Neural Networks with Bandpass Filtering, 2018.
Image Super-Resolution
Image super-resolution is the task of generating a new version of an image with a higher resolution and detail than the original image.
Often models developed for image super-resolution can be used for image restoration and inpainting as they solve related problems.
Datasets often involve using existing photo datasets and creating down-scaled versions of photos for which models must learn to create super-resolution versions.

Example of the Results From Different Super-Resolution Techniques.
Taken from “Photo-Realistic Single Image Super-Resolution Using a Generative Adversarial Network”
Some papers include:
- Photo-Realistic Single Image Super-Resolution Using a Generative Adversarial Network, 2017.
- Deep Laplacian Pyramid Networks for Fast and Accurate Super-Resolution, 2017.
- Deep Image Prior, 2017.
Image Synthesis
Image synthesis is the task of generating targeted modifications of existing images or entirely new images.
This is a very broad area that is rapidly advancing.
It may include small modifications of image and video (e.g. image-to-image translations), such as:
- Changing the style of an object in a scene.
- Adding an object to a scene.
- Adding a face to a scene.
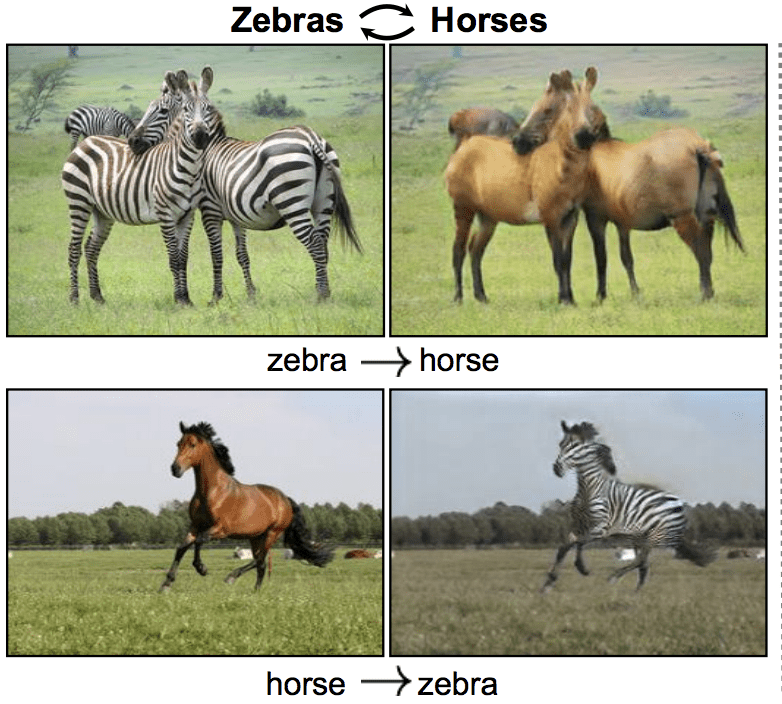
Example of Styling Zebras and Horses.
Taken from “Unpaired Image-to-Image Translation using Cycle-Consistent Adversarial Networks”
It may also include generating entirely new images, such as:
- Generating faces.
- Generating bathrooms.
- Generating clothes.
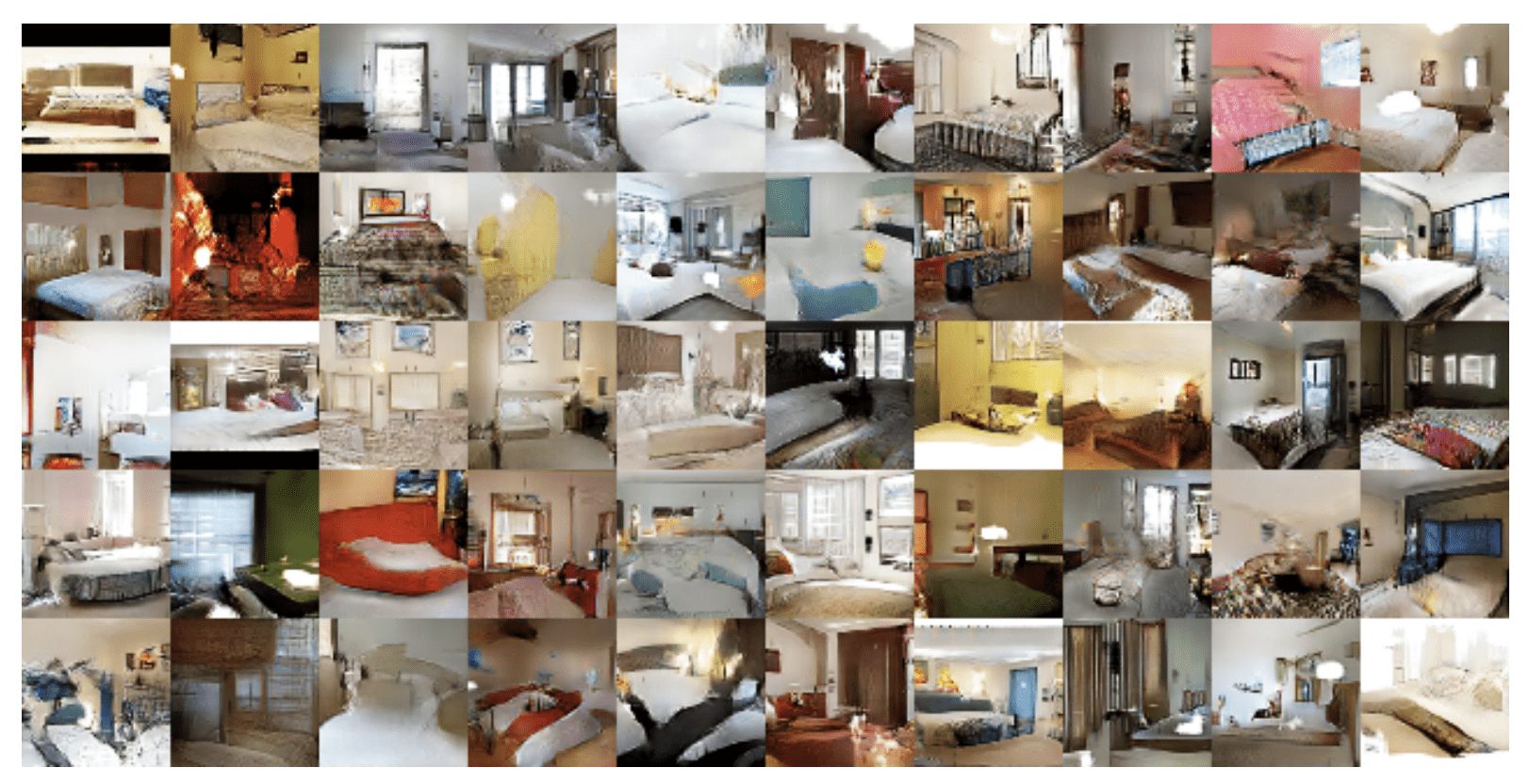
Example of Generated Bathrooms.
Taken from “Unsupervised Representation Learning with Deep Convolutional Generative Adversarial Networks”
Some papers include:
- Unsupervised Representation Learning with Deep Convolutional Generative Adversarial Networks, 2015.
- Conditional Image Generation with PixelCNN Decoders, 2016.
- Unpaired Image-to-Image Translation using Cycle-Consistent Adversarial Networks, 2017.
Other Problems
There are other important and interesting problems that I did not cover because they are not purely computer vision tasks.
Notable examples image to text and text to image:
- Image Captioning: Generating a textual description of an image.
- Image Describing: Generating a textual description of each object in an image.
- Text to Image: Synthesizing an image based on a textual description.
Presumably, one learns to map between other modalities and images, such as audio.
Further Reading
This section provides more resources on the topic if you are looking to go deeper.
Survey Papers
- Object Detection with Deep Learning: A Review, 2018.
- A Survey of Modern Object Detection Literature using Deep Learning, 2018.
- A Survey on Deep Learning in Medical Image Analysis, 2017.
Datasets
- MNIST Dataset
- The Street View House Numbers (SVHN) Dataset
- ImageNet Dataset
- Large Scale Visual Recognition Challenge (ILSVRC)
- ILSVRC2016 Dataset
- The PASCAL Visual Object Classes Homepage
- MS COCO Dataset.
- The KITTI Vision Benchmark Suite
Articles
- What is the class of this image?
- The 9 Deep Learning Papers You Need To Know About (Understanding CNNs Part 3)
- GAN paper list and review
- A 2017 Guide to Semantic Segmentation with Deep Learning.
References
Summary
In this post, you discovered nine applications of deep learning to computer vision tasks.
Was your favorite example of deep learning for computer vision missed?
Let me know in the comments.
Do you have any questions?
Ask your questions in the comments below and I will do my best to answer.
The post 9 Applications of Deep Learning for Computer Vision appeared first on Machine Learning Mastery.